SIGNAL TIMING UNDER SATURATED CONDITIONS
Guidance
About This Document
This document is organized to provide guidance as a summary of the material that follows. The subsequent chapters provide detailed documentation of the work performed that lead to this guidance. It should be noted that some guidance is supported by results from interviews with a panel of experts, some is based on research conducted as part of this project, and some is a synthesis of practice recommended by the study team. The text will attempt to make these sources clear so that the reader can reasonably assess the reliability of the guidance.
The project was organized in three parts. The first part (Chapter 1) was a literature review on the general subject of signal timing in congested conditions and on the characterization of saturation. The literature review continued with a discussion of the NCHRP 3-66 project, which was anticipated to relate to this project to a greater extent than actually proved to be the case. The literature review was expanded slightly to include a review of work done on the subject of cycle length, long green times, and saturation flow. Because that work is directly related to the work of the first section of Chapter III, it is included there.
The second part of the project (Chapter 2) identifies methods used by acknowledged experts to address congested conditions using signal timing. To collect data and information through interviews, the research team developed a framework for categorizing methods by their underlying objective; this is a dominant theme of the Guidance section. The interviews identified a range of methods that might be used in congested conditions. Some are in the form of principles to understand, and some are specific approaches to signal timing. All of the proposed methods are limited to solutions that can be implemented at the intersection level. Network-level solutions are being explored in the related NCHRP 3-90 project, which is underway at the time of this writing, and which will incorporate the findings of this project.
The third part of this project (Chapter 3) involved simulation and field
studies to evaluate some of the methods that emerged from the review of
the state of the practice. Not all methods could be researched within the
context of this project, but focus was given to those issues that are
often misunderstood or that have an extraordinary impact on operations in
the opinion of the research team. These studies are documented in Chapter
3.
This Guidance section will start with a discussion of objective functions as the basis for decided what tools to implement in the congested case. Understanding how the methods relate to the objectives will help avoid self-defeating interactions. The authors recommend that all practitioners consider their objectives when faced with a congested scenario, before deciding what to do.
Following the discussion of objectives, this Guidance chapter will present the strategies that emerged from the interviews and from the research that was conducted.
Objectives
Practitioners are trained in methods, and those methods are designed to achieve a specific goal (not always perfectly). For example, one might time traffic signals to maximize progression, minimize delay, minimize cycle failures, minimize fuel consumption, maximize throughput, manage the location and size of queues, and so on. Not all signal timing methods are characterized in terms of their objective, with the result that practitioners sometime employ methods inappropriate to the situation at hand. It is the recommendation of the researchers that practitioners carefully consider their objectives. To assist with this recommendation, the researchers have characterized objectives in the following sections.
Traditional Objectives
Minimizing Cycle Failures
This objective seeks to minimize the frequency that a traffic signal does not serve all the waiting cars during the green period.
The Yale Bureau of Highway Traffic, which was the original traffic engineering study program at the post-graduate level in the U.S., promoted a technique for determining cycle length and green times based on mean arrivals. Once mean arrivals were known, an assumption that arrivals were random could be used as the basis for estimating the number of arrivals that would only be exceeded a small percentage of the time (say, 5%). The Poisson Distribution was used to make this estimation, and this method has come to be known as the Poisson Method. Because it seeks to provide green times long enough to accommodate nearly all arriving flows, it demonstrates the objective of minimizing cycle failures.
Cycle failures occur when the green time is not long enough to serve all the cars that were waiting at the start of green. In and of themselves, cycle failures are not evidence of congestion. But when the remaining queue at the end of green, which we call the residual queue, grows over a series of cycles, most experts will agree that the conditions have become congested.
Minimizing cycle failures is not the same thing as minimizing residual queuing. As volumes increase, the 95th percentile arrival will become too large to accommodate in a cycle, and the method will no longer converge on a reasonable solution. Also, the method looks only at expected arrivals, not at actual arrivals, and thus uses a cycle that is longer than needed much of the time. Actuation at a non-coordinated signal might overcome that problem to some extent, if the detectors are carefully placed and the actuated control logic so optimized (see NCHRP 3-66 for more guidance on this issue).
Thus, the Poisson Method, and any other method with the objective of minimizing cycle failures, is only appropriate in light traffic conditions where the motorist expectation is to be served on the next available green.
Maximizing Performance Measures
In this objective, the methods used seek to increase some positive performance measure or decrease a negative performance measure to the extent possible.
To address the limitations of the Poisson Method, researchers started to develop tools that would achieve more attainable objectives in heavier traffic conditions. These tools characterize performance measures using analytical or empirical simulation models, and adjust timing systematically to optimize those performance measures.
The performance measures are typically one of two possibilities:
- Progression, where coordination timings are adjusted to maximize the opportunity to drive through successive signals on a green light.
- Delay and stop in some combination, as a general performance measure.
Both of these have problems in the presence of growing residual queues. Progression assumes that vehicles can move unimpeded down the street as long as the signal timings are appropriate, and usually ignore the possibility of a residual queue providing that impedance.
Delay is also difficult to characterize in the congested regime. Queues form and grow when demand exceeds capacity, and the delay caused by the queue depends on its length. Thus, longer queues mean more delay. If queues are growing, the delay becomes a function of how long one collects the data, rather than a measure of performance that can be optimized.
Thus, delay minimization and progression, as tools for practical signal timing, are limited to moderate traffic conditions free of growing residual queues.
In the research reported in Chapter III, the researchers define "demand exceeds capacity" more carefully as that point at which increases in the offered load at the intersection can no longer be served. That serves as the definition of saturation, and it is visible in the field by growing residual queues. Thus, the presence of repeated residual queues is the symptom that should encourage the practitioner to move to a different objective.
Maximizing Throughput
In this work, the researchers develop the objective of maximizing throughput, or the number of cars actually served by the intersection, with respect to the cars presented to the intersection (the load).
A cycle failure causes a residual queue, which adds to the demand to be served on the next cycle, in addition to new arrivals. Even if the new arrivals are few enough to be served by the next green period, the residual queue from the previous cycle might cause a cycle failure by consuming green time needed by the freshly arriving flow. Thus, normal variation in traffic that causes occasional cycle failures makes it more difficult for the signal to recover without a change in timing strategy. If the variation in demand provides sufficient reductions in demand such that the residual queue can just clear before growing again, then we might call this condition intermittent congestion. Any increase in average demand will prevent this recovery. Periods of several failed cycles followed by a cycle or two that clear, followed by more failed cycles provide a useful indication that the capacity of the current operation has been reached, and the operation is crossing into the congested regime.
The residual queue prevents progression, so progression is no longer a meaningful objective as demand approaches capacity at key intersections in the system (except perhaps for carrying traffic away from a bottleneck intersection). Also, delay becomes difficult to define. Intersection delay can be characterized by the area between the arrival flow curve and the departure flow curve, as shown in the Figure G-1.
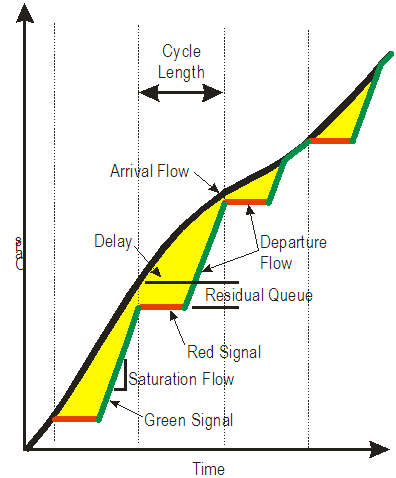
Figure G-1. Delay resulting from arrival and departure flows at an intersection.
(It should be noted that the figure suggests that the departure flow maintains saturation flow either until the queue dissipates or the light turns red, and this research will show that this is an assumption that does not hold for long green times when the approach traffic contains turning traffic that cannot reach turn lanes. The shape in the figure are idealized for clarity.)
When demand exceeds capacity and the residual queues no longer clear, the delay grows as the queue grows and the arrival and departure flow curves in the intersection diverge.
Another way to define this point is to compare the demand of the intersection to the throughput by counting cars going into the intersection versus cars coming out of the intersection. As the queue grows, the cars coming out reach a maximum that is constrained by the available green time, while the arrives cars are not constrained. Figure 3-10, later in the this document, shows a typical relationship between the demand and throughput, where demand is characterized as offered load and throughput as served load. In this work, we have defined capacity as the point where throughput no longer increases with increased demand. Demand and throughput are traffic engineering terms that are similar to the optimization terms of offered and served loads, and we use these terms interchangeably in this report.
When an intersection has residual queuing that is just beginning to grow over time, the maximum throughput of the intersection has been reached.
The practitioner should then first attempt to improve the maximum throughput to the extent possible by implementing one of a range of signal timing strategies (or by avoiding one of several signal timing mistakes). These strategies and mistakes are the subject of this research and the next section will describe them.
Maximizing throughput has the aim of minimizing queue formation to prevent congestion. At some point, however, no revision to the signal timing will increase maximum throughput, and the queues will continue to grow until demand diminishes. The practitioner's objectives will need to shift again, this time to one of queue management.
Queue Management
The queue management objective seeks to use signal timing to allow queues to form where they do the least damage.
When growing residual queues can no longer be relieved by maximizing throughput, then the practitioner's only choice becomes arranging the operation of signals within a network to prevent the queues from multiplying the problem by creating capacity constraints of their own. This usually means constraining capacity upstream from a bottleneck at locations where queue storage will not cause network gridlock, or where safety problems might occur as a result of queue formation.
If throughput maximization is a curative approach, then queue management can be considered a palliative approach with the objective of treating symptoms rather than seeking a cure.
Strategies
In this project, the researchers adopted two approaches to identifying effective methods for optimizing signal timing in congested conditions to satisfy the objectives of optimizing throughput or managing queues. The first of these was to form a panel of acknowledged expert practitioners. The members of the panel were interviewed to identify examples from their experience that illustrated techniques that they used. These techniques were then evaluated to determine their underlying objective (which was mixed in some cases). Some were used as the basis for further consideration. In all cases, the experts knew what they were attempting, even if they did not articulate it, and identifying their objective helps to explain their approach. We recommend that practitioners consider their objective when addressing congestion at an intersection. All the experts first attempted to cure the congestion by maximizing throughput, and when that was not adequate they employed queue management strategies.
Next the researchers attempted to evaluate several approaches identified during the interviews that warranted further research. Some of these approaches can be adequately explained and recommended without further research, but some issues and observations were inconsistent with the observed beliefs of some practitioners, and therefore warranted further research. Those issues were evaluated using simulation and, in one case, field studies.
Guidance from Experts
Project researchers conducted a series of interviews with leading practitioners from around the country (See Chapter II, The State of the Practice for details). The interviews were not structured, and the intent was to let the experts lead the discussion as a means of identifying underling principles, objectives, and priorities. Each interviewee also provided a case study, and many of these are documented in Chapter II. This section will summarize those interviews in the form of guidance for practitioners.
Common Evaluation Themes
Experts were not generally interested in defining the point of saturation in a precise or scientifically elegant way, but were rather interested in conditions that would justify a change in strategy or more detailed adjustment to operation on the ground. When pressed, the experts generally agreed with a definition of saturation as when maximum throughput is exceeded, or when residual queues grow. These were reported as being triggers for action, and some tightened their action level to growing queues that caused more general network problems. When discussing methods for evaluating congested locations, some common themes emerged. These included:
- Inadequacy of existing evaluation tools
- Inadequacy of existing optimization tools
- The necessity for performing evaluations on the ground by direct observation.
- The necessity to devote sufficient observation to fully understand the problem and the effect of candidate solutions.
Evaluation tools that were generally considered to be inadequate included capacity methods (as a means of identifying maximum throughput) or any computer-based models short of some (and only some) microscopic simulation. For the experts, the determination of congested was based on a growing queue problem, and that was determined solely by direct observation.
The experts also generally did not use signal timing optimization tools to help solve a congestion problem. Most reported that those methods seemed insensitive to even the existence of growing residual queues, let alone the input of that condition into the optimization objective. This impression is supported by a cursory review of available tools, which based signal timing on delay or some other performance measure, or on balancing the saturation level of the various movements at the intersection. No existing popular tools could be identified by the experts that optimized specifically to maximize throughput or to manage queues. The recommendation to practitioners is to avoid depending on signal timing optimization tools to provide solutions for situations where growing residual queues indicate that the objective should shift to maximizing throughput.
In all the case studies reported here, the experts invested considerable time in observing, understanding, and addressing the problem. Resources are constrained in most agencies, and this devotion of attention reflects the priority given by experts in addressing points of congestion. In many cases, the expert spent days of observation before formulating a plan, and then days of implementation and operation to refine and evaluate the solution. The recommendation for practitioners emerging from these examples is to invest the time the most where the congestion is the worst, because that is where limited resources may do the greatest good. Another recommendation is to commit the most experienced experts available in the organization to observe and address the worst problems.
Throughput Strategies
A number of general techniques presented by the experts could be categorized as strategies aimed at maximizing throughput. These include:
- Work back from downstream bottleneck
- Run close intersections on single controller
- Improve lane utilization
- Lag heavy left turns
- Find the right cycle
- Serve heavy movements more than once in a cycle
- Consider effect of buses
- Minimize effect of pedestrian movements
- Seek all possible available green time, even if esoteric signal controller features are required
- Consider congested and uncongested movements separately
- Prevent actuated short greens
These can be broadly divided into three categories:
- Making best use of the physical space provided in the intersection
- Making best use of green time in the cycle
- Minimizing the negative effects of other influences.
Several of these were studied in greater detail in later project phases, including running close intersections on a single controller, considering the effect of buses, and, most important of all in the opinion of the researchers, finding the right cycle. These will be summarized further down in this Guidance section and reported in more detail in later chapters.
All of these methods can be readily implemented in existing signal control equipment, though a more straightforward phase reservice capability was mentioned as desirable. Phase reservice is a feature that allows one phase to be served more than once in a cycle. Chapter II shows an example of how this might be done with a very simple eight-phase controller. Phase reservice serves both a throughput objective and a queue management objective. By serving congested movements twice in a cycle, uncongested movements will consume less of the effective cycle without violating required minimum green periods or other constraints. The example in Chapter II shows how minor movements, by being served only once for every two times the congested phases are served, improve the percentage of the cycle devoted to the congested movements. This is the single most effective strategy for relieving one congested movement in cases where the uncongested movements are constrained to longer green times than needed by cars or to exclusive phase protection.
A closely related principle was making use of any feature of the controller that could serve to increase the percentage of the cycle devoted to the congested movements. Several of the experts agreed that they were prepared to use even extremely esoteric combinations of controller features to that end, though one expert countered that attitude by avoiding techniques that could not be understood or maintained by maintenance technicians.
Most of the experts warned that actuation could defeat the objective of maximum throughput. They did not mention that sluggish extension timings could cause greens to be extended for traffic of lower flow rather than serving higher flows on other movements, though the experts generally supported sharp actuated timing to minimize extension, and other experts worked in the context of coordination when addressing congested signals. They did, however, make extensive use of actuation features to maximize the amount of the cycle that would serve maximum flow over the stop line. They also warned of congested movements being cut short because of a single sluggish vehicle such as a truck, with the observation that even one untimely gap out could significantly increase a residual queue such that it would take many cycles to recover.
Queue Management Strategies
When throughput cannot be maximized sufficiently to control the queues at a congested intersection, the experts suggested a range of techniques that are aimed at managing the resulting queues to minimize the propagation of congestion within the network. They include:
- Reduce minor splits to encourage diversion (usually doesn't work)
- Run close intersections on single controller
- Balance queue for conflicting approaches
- Prevent queue from multiplying congestion
- Meter traffic into bottlenecks
- Prevent backing queues into bottlenecks
Some of these also appeared as throughput strategies, such as running close intersections on a single controller. This approach should be given priority when carrying queues through two intersections is too important for minimizing congestion to be relegated to a low-priority controller function such as coordination. Coordination can be overridden by most controller functions, including preemption, pedestrian and minimum greens, and (in some cases) maximum greens. Also, coordination implies cycle-based patterns that will usually change during the day, resulting in transitions during which coordination may break down temporarily. When two intersections are run from a single controller, however, the relationship between them can be built into the phasing design and therefore maintained even during high-priority controller functions such as preemption. Diamond interchanges provide an example that will be explored further down.
Demons
The experts also identified conditions or situations that work against optimizing for throughput or queue management. These include:
- Longer and longer vehicle clearances (especially red clearances)
- Longer pedestrian clearances
- Too much dependence on detection
The clearance issue is especially timely. The 2003 edition of the Manual on Uniform Traffic Control Devices has increased the time required for pedestrian crossings to allow pedestrians to cross the entire traveled way, instead of just to the center of the far lane. The FHWA has proposed for the next edition of the MUTCD to reduce the assumed walking speed used for calculating the pedestrian clearance time from 4 feet/second to 3.5 feet/second. If adopted, this will change pedestrian crossing times yet again.
More subtle, though, are increases in red clearance. Many agencies have been ratcheting up red clearances for a variety of reasons. These are justified on the basis of having only a small effect on signal timing, but with the expectation of a larger effect on safety. The small effect on signal timing is based on an often false assumption, however. The assumption is that the clearance is provided by reducing the green time for the phase being cleared.
This is not the case, however, at intersections where one movement is congested. The other movements will have been reduced to an arbitrary minimum green, either constrained by the agency's policy on minimum greens or by pedestrian clearance times. Thus, the green times of the uncongested movements cannot be further shortened—they are as short as they are allowed to be. An increase in red times for these movements do not take away from the green time for these movements, but rather accumulate around the signal cycle to the congested phases, where green time that can be shortened is available. Thus, even a modest increase in clearance of only, say, one second in each phase might result in shortening a congested green by four seconds, which further might reduce throughput by, say, 8%, assuming a 50-second green in a 100-second cycle. Thus change in clearances, which was assumed to be insignificant, can result in a significant reduction in green time just where the green time is most needed. The true consequences of these clearance interval decisions should be considered before those decisions are made and implemented.
Controller Features
The experts interviewed for the project described a range of signal controller features that they employed to address some aspect of maximizing throughput or managing queues. These included:
- Phase Reservice, with the general recommendation to serve congested movements more often in the cycle, or alternatively to serve movements that are too lightly traveled to deserve their minimum greens on alternate cycles.
- Minimize Ped Effects
- Let Peds override forceoffs, or
- Use additional Max in lieu of forceoffs by programming max time for the normal coordinated green time and accommodating ped times within the coordination timings. With max not inhibited, the green will be routinely short because the phase will max out, except when a ped call is being served, in which case the ped intervals will override the max, all within the context of the coordinated plan.
- Use special logic, such as terminating the side street if remaining cars are right turns that can be served during complementary left turn
- Simultaneous Gaps—don't let phase extend again if detection is received during green rest
- Dual Entry so that late-arriving cars on the opposing minor approach won't cause a delayed start of green
- Volume-Density variable gap to prevent actuated short greens, such as those that might be caused by a truck accelerating from stop too slowly.
Cycle Length and Green Time
The experts noted that flow seemed to decrease as green times extended beyond about 30 seconds, even when fed by an arbitrarily long queue. Project researchers explored this issue by conducting a field test at a site in Northern Virginia, as reported in Chapter III. That work has led to several conclusions:
- Saturation flow does not decline in lanes unaffected by cars leaving the lane, but
- Lanes adjacent to turn lanes do see a significant reduction in saturation flow as turners depart the through lane for the turn lanes. At the study site, a 12% turning volume resulted in a significant reduction in throughput for the through movement. The reduction was sufficient to reduce the overall throughput of very long cycles, as indicated by simulation studies based on the field site.
The guidance for practitioners is that on approaches with turn lanes, the green time and cycle should be kept short enough to serve only vehicles queued up to the upstream entrance to those turn lanes. That way, the green time serves only through cars, with the assumption that turning cars will be served during other phases (as with left turns) or in lanes that can move with other phases (as in both left and right turns). In many locations, this green time may result in unacceptably short minor movements or in violations of required minimum intervals, and in those cases, the shortest cycle that does not cause those problems may provide the greatest throughput.
Practitioners are encouraged to abandon the commonly held belief that long cycles are naturally more efficient because lost time is reduced as a percentage of the cycle. This effect was not confirmed, and other effects were found to be much more dominant.
Buses
One effect that emerged from the experts was that cycle length was related to bus capacity. The researchers tested this concept using simulation of three intersections along a downtown one-way street. The simulation was formulated to eliminate the effect of cycle length on progression, and the upstream intersection metered flow into the network by providing less green time for the main street than downstream intersections. Thus, the simulation was sensitive only to the effects of buses.
The simulation shows that changes in bus volumes did not significantly affect the throughput of the street. But the size of the residual queue was significantly affected by changes in the cycle length. The conclusion is that the interaction of buses and the cycle length can have a significant effect on congested flow, and that it is that interaction with cycle length, not bus volumes per se, that have the effect.
The cycle length that performed the best was the shortest cycle that could reliably serve at least one bus arriving during that cycle. The cycle that performed the worst was the longest cycle that could not reliably serve more than one bus. Thus, for buses that stop on a congested approach and that require a typical 20-second loading and unloading time, the best cycle is about 40 seconds and the worst cycle is about 80 seconds. The cycles below 40 and the cycle above 80 were noticeably worse in their effect on the number of cars in the network (and therefore the total delay) than the cycles from 40 to 70 seconds. The guidance is therefore to use the shortest cycle on congested approach that carry significant bus demand, and that have a stop on the approach, that will reliably serve at least one bus per cycle. In the simulation, that was shown to be about twice the normal bus loading and unloading time. The cycles from that value up to about 50% longer provide the best operation in this scenario.