Approaches to Forecasting the Third Performance Management Rulemaking (PM3) Measures for Target Setting
Chapter 5. Machine Learning Model-based Forecasts of Targets
The Virginia Department of Transportation (VDOT) developed an analytical procedure to provide guidance in setting targets for the Interstate Travel Time Reliability Measure. It is hoped that the methodology can be extended to the other PM3 measures, as well as other travel time-based measures used by VDOT. The steps in the VDOT procedure are described below.
- Calculate Current Interstate Reliability. VDOT used the NPMRDS data and the recommended procedure for calculating both the metrics (LOTTR) by TMC and time periods, as well as the overall system reliability measure. Virginia’s Interstate Travel Time Reliability Measure in year 2019 was 83.55 percent.
- Examine Patterns in the 80th and 50th Percentile Travel Times. To gain a deeper understanding of the Interstate system reliability measure, VDOT undertook a detailed analysis to explore the relationship between the 80th and 50th percentile travel times, the foundation of the metrics, and resulting measure. Figure 6 shows an example of the analysis that included multiple congested Interstates. The ratio between the 80th and 50th percentiles is shown in the bottom graph. Three regimes are shown:
- “Reliable” where average speeds are high and the ratio is close to 1.0.
- “Unreliable” where speeds are transitioning from high to low and the ratio is above 1.5.
- “Reliably Congested” where speeds are low and the ratio is within a few points of 1.5.
While the term ”Reliably Congested“ may seem contradictory, it is a function of the two percentiles chosen for the LOTTR metric. In situations where a roadway segment experiences congestion just about every peak period, the 50th percentile (a measure of central tendency) is relatively high and not that greatly different from the 80th percentile. If the upper bound is changed to a higher percentile (e.g., 95th percentile travel time), it is possible that the same roadway would just be deemed ”unreliable.“
- Develop a Model to Predict Future Interstate System Reliability. A machine learning model using the Random Forest algorithm was developed to predict the 80th and 50th percentile travel times on TMCs. There were 30 independent variables selected, including geometric features, weather conditions, incident characteristics, work zone characteristics, and traffic demand.18 The model demonstrated very small (less than 1 percent) error when it was used to “predict” the reserved validation dataset.
- Forecast Key Independent Variables for Future Condition. The following forecasts are made in order to apply the model:
- Number of lanes and acceleration/deceleration lane extensions: based capacity improvements in the on Six-Year Improvement Program Project Types.
- Future year v/c ratio and future year crashes based on forecasted number of lanes, projected average annual daily traffic (AADT) using yearly growth factors, and safety performance functions for crashes.
The results of applying the model are shown in figure 7.
- Extend the Model to Include Other Reliability Measures. The PM3 System Reliability measure is just one of several potential reliability measures. Other measures should be considered as well that do not have the limitations of the System Reliability measure:
- Long time periods (4/6/10 hours) do not reflect peak-hour travel conditions and can “dilute” reliability measurements.
- Seasonal fluctuations in travel demand are not addressed.
- The pass/fail nature of the System Reliability measures does not indicate how strongly a roadway is reliable or unreliable.
The same methodology as used for the System Reliability measure can be used for other travel time-based measures, including reliability.
Figure 6. Chart. Example of reliability along a facility: I-66 Eastbound AM peak (6 a.m.–10 a.m.).
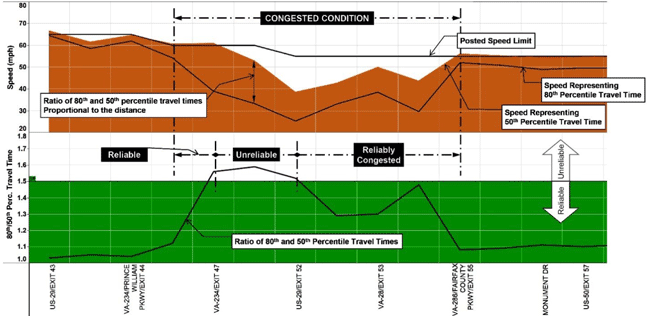
(Source: Virginia Department of Transportation.)
Figure 7. Chart. Interstate travel time reliability measure prediction—statewide.
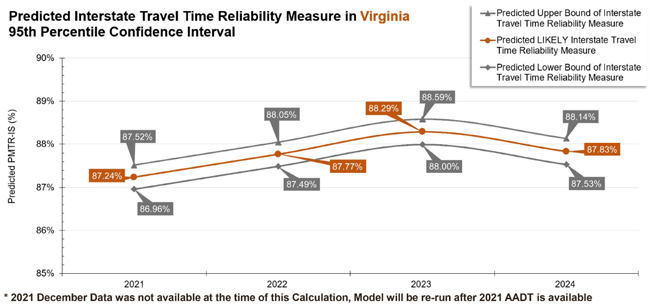
(Source: Virginia Department of Transportation.)