Operations Benefit/Cost Analysis Desk Reference
Chapter 3. Operations Strategies and their Impacts (Agency, User, and Societal Benefits)
Definitions of Operations Strategies Covered in this Reference
This Desk Reference and the supporting TOPS-BC application provide guidance regarding a wide range of TSM&O strategies. Additionally, in many cases, the guidance provided can equally be applied to nonoperational strategies; however, the focus of this guidance is specifically on TSM&O strategies.
The TSM&O strategies covered in this guidance represent a mix of long established, as well as recently emerging technologies. The strategies include investments requiring the deployment of physical systems and hardware, as well as nonphysical improvements, such as enhancing interagency coordination. In general, the strategies are categorized into three groups.
- Operations Strategies – These strategies involve the deployment of physical infrastructure to the roadside or transit assets, and are intended to provide direct impact on transportation system performance through their operation. These strategies are often highly visible to the traveling public and include a wide range of deployments, such as traffic signal coordination, freeway service patrols, 511 traveler information systems, HOT lanes, and DMS, among many others.
- Supporting Infrastructure – Supporting infrastructure includes those backbone capabilities that serve to support and enhance the functioning of the roadside Operations strategies. This backbone infrastructure includes items, such as traffic detection and surveillance, communications, and traffic management centers. Often, by themselves, these strategies have no direct intrinsic benefits. The benefits derived from these strategies are the improved performance of the roadside Operations strategies enabled by their deployment. For example, a traffic surveillance camera by itself has little direct benefit. The benefit is derived from the manner in which the information is used (e.g., when the surveillance from the camera is used by operators to implement different signal timing patterns, or detect and respond to an incident faster). Therefore, the measure of benefit of these supporting infrastructure components is often measured by estimating the improved efficiency of the roadside components supported by the backbone infrastructure, rather than attempting to directly estimate the benefits of the supporting infrastructure itself.
- Nonphysical Strategies – These strategies represent items intended to improve the efficiency and effectiveness of the TSM&O systems and includes strategies, such as improved interagency coordination; the development of advanced operational plans (e.g., evacuation plans); or system integration. While there certainly can be benefits of these activities and there are often costs, there typically is little or no new physical equipment or roadside components. Similar to the supporting deployments, the benefits derived from these strategies are typically estimated by assessing the improvement in effectiveness of the existing roadside components provided by the strategy or activity.
These categories and the strategies belonging to the various groupings are discussed in the following sections.
Operations Strategies
The following are operations strategies discussed in this Desk Reference and included in the TOPS-BC guidance:
- Arterial Signal Coordination – This strategy involves the coordination of traffic signal timing patterns and algorithms to smooth traffic flows – reducing stops and delays and improving travel times. This strategy can be implemented on a small corridor, a limited grid, or regionwide in aggressive deployments. The sophistication of the timing coordination can also vary from simple preset timing programs to more advanced traffic actuated corridor systems, to fully centrally controlled applications.
- Arterial Transit Signal Priority – This strategy involves installing transponders on transit vehicles to communicate with the traffic signal systems. Traffic signal timing patterns are adjusted when a transit vehicle is detected approaching the intersection – either providing an early green phase or extending an existing green phase – to allow the transit vehicle to reduce stop delays through the corridor, improving travel times and travel time reliability (maintaining schedules).
- Transit AVL – This strategy involves equipping transit vehicles with transponders and communication capabilities to allow for the real-time tracking of the vehicles by the transit agency. This information is used to better manage the fleet and improve schedule maintenance, thus improving travel time reliability for users and asset use efficiency for the agency. The information generated by the AVL is also often used to support real-time transit traveler information for users.
- Ramp Metering – This strategy involves the placement of a traffic signal on freeway on-ramps to meter the flow of traffic entering the mainline facility and smoothing the flow of traffic in the merge area. Ramp metering may be implemented with minimal cycle lengths designed to simply break up platoons of vehicles entering the facility to smooth the merge operations, or may be operated more aggressively with longer cycle lengths designed to hold traffic on the on-ramp to maintain lower volumes and higher speeds on the mainline facility. Ramp meters may be deployed at single isolated locations, or may be deployed regionwide and are intended to improve merge operations and reduce bottlenecks at on-ramp locations, thus improving corridor travel times and safety. Similar to arterial signal systems, the sophistication of the timing patterns may be determined according to preset, traffic actuated, or centrally-controlled patterns.
- Traffic Incident Management (TIM) – These strategies are often divided into several substrategies that may be combined to create a coordinated system. The benefits of these systems include a reduction in incident related delay (and associated fuel use and emissions impacts), and can include safety benefits by allowing for the faster dispatch and response of emergency personnel and assets to injury accidents. The TIM substrategies include the following:
- Incident Detection and Verification – Involves the implementation of surveillance, detection, communications, and algorithms to enhance the monitoring of the transportation system to more quickly detect the occurrence of incidents and provide more information to system operators to verify the location and severity of the incident, so that an appropriate response plan may be developed and implemented.
- Incident Response – Involves the improved development, communication, and implementation of response plans through coordinated response strategies and computer-aided dispatch (CAD) systems.
- Freeway Service Patrols (FSP) – Involves prepositioned or roving “highway helper” vehicles designed to quickly respond to system incidents and mitigate the situation. These vehicles may also provide first responder capabilities for more series crashes or incidents until more appropriate emergency assets and personnel arrive on scene.
- Pretrip Traveler Information – These strategies provide single mode or multimodal information to travelers prior to the initiation of their trip enabling the user to make better informed decisions on the route, mode, and timing of their travel. Often developed as regional 511 programs, these systems may deliver the information content via telephone, Internet, kiosks, or mobile devices. The enhanced information provided by these systems may also be distributed by existing media outlets (e.g., television or radio).
- In-route Traveler Information – These strategies may provide a variety of information (e.g., travel times, mode change opportunities, construction work zone information, incident warnings, and alternative route recommendations) to travelers already using the system, allowing the travelers to make more informed decisions on travel route and mode choice. Traditionally provided through DMS or changeable message signs (CMS), HAR, and through distribution by traditional media outlets (radio), the channels for distribution have been expanded in recent years to increasingly include in-vehicle and mobile devices.
- HOT Lanes – Allows SOV to pay a toll to use underutilized HOV lane capacity. These systems most often utilize an in-vehicle transponder to determine lane usage and assess tolls. The tolls charged may vary according to time-of-day schedules, or may be dynamically assessed in response to traffic conditions and available HOV lane capacity.
- Speed Harmonization – Involves the implementation of variable speed limits and the communication of those limits through roadside signs. The speed limits are modified according to congestion levels to lessen stop-and-go conditions and lower the speed of vehicles as they approach downstream bottlenecks. The primary benefit of these emerging systems is improved safety.
- Hard Shoulder Running – Involves allowing vehicles to travel on the shoulder facilities of roadways often for isolated sections of roadway or limited times of operation. The availability of the shoulder for use is often communicated through the use of overhead gantries or roadside DMS.
- Work Zone Management – Involves the coordinated implementation and use of pretrip (e.g., 511 web-based applications) and en-route (e.g., DMS and HAR) traveler information, along with construction traffic management and alternative construction work hours planning to mitigate the congestion related to construction work zones.
- Travel Demand Management – Includes a number of strategies that may be employed to lessen travel demand (number of trips) associated with work commuting traffic. These strategies may include employee-based subsidies for alternative mode use or alternative work hours, or employer-based investment in vanpools or alterative work campuses to lessen the number of trips made by their employees.
Supporting Infrastructure
Supporting infrastructure includes those strategies that provide the backbone infrastructure that enables the effective operation of the roadside components. The benefits from these supporting infrastructure implementations are often measured by estimating the effectiveness and efficiency gains to the roadside components directly supported by the strategy. Supporting infrastructure discussed in this Desk Reference includes: (Since many of the Supporting Infrastructure components have no direct benefits – their benefits are estimated through the roadside Operations Strategies supported by their implementation – they are not included as strategies available for benefit estimation in the TOPS-BC application. However, the ability to estimate the life-cycle costs of these components is provided within TOPS-BC. Section 5 of this Desk Reference provides additional detail on structuring the estimation of benefits of these Supporting Infrastructure strategies within a benefit/cost analysis framework.)
- Traffic Detection and Surveillance – Involves the implementation of traffic detection (e.g., loop detectors, radar detectors, acoustic detectors) and/or camera surveillance systems; and the development of associated data and analysis systems to provide the ability to monitor real-time conditions on the transportation system. This data is often used to support and enhance the capabilities of many Operations Strategies, including traffic signal coordination, ramp metering, incident management and Active Traffic Management (ATM), and traveler information systems.
- Traffic Management Centers – Include the regional traffic and transit management centers, as well as the smaller subregional centers, required to monitor, coordinate, and operate the deployed Operations Strategies.
- Communications – Include the landline and wireless communication networks required to coordinate and operate the Operations Strategies implemented in a region.
Nonphysical Strategies
Nonphysical Strategies are intended to improve the efficiency and effectiveness of the deployed TSM&O systems. The FHWA Integrated Corridor Management (ICM) initiative is an example of a project that heavily leverages many nonphysical strategies. This category includes strategies such as:
- ATDM – The dynamic management, control, and influence of travel demand, traffic demand, and traffic flow of transportation facilities. Through the use of available tools and assets, traffic flow is managed and traveler behavior is influenced in real time to achieve operational objectives, such as preventing or delaying breakdown conditions, improving safety, reducing emissions, or maximizing system efficiency. Under an ATDM approach, the transportation system is continuously monitored. Using archived data and or/predictive methods, actions are performed in real time to achieve or maintain system performance.
- System Integration – Involves the integration of two or more systems to allow for the improved exchange of data between the systems and/or the coordinated operation of the systems. For example, a traffic signal coordination system could be integrated with a traffic incident management system to provide additional capacity on parallel diversion routes when incidents occur on a freeway facility.
- Interagency Coordination – Involves the exchange of information and/or agreements allowing for the joint operation of various strategies across different agencies or jurisdictions.
- Regional Concepts for Transportation Operations (RCTO) – The development of regional concepts of transportation operations involves the coordination of the various stakeholders responsible for operating one or more components in order to develop sets of policies, procedures, and operating parameters that may be implemented according to specific identified conditions to improve the efficiency and effectiveness of Operational Strategies during those conditions. The development of a coordinated strategy for operating strategies during a regional evacuation would be an example of a regional concept of transportation operations that might be analyzed using benefit/cost analysis. The FHWA Integrated Corridor Management (ICM) initiative serves as another example as the Concepts of Operations developed in support of this initiative represent RCTO.
What are the Impacts and Benefits of Operations Strategies?
The operations strategies, discussed in Chapter 3 above, all impact the transportation system, traveler behaviors, and agency operations in different ways. For example, implementation and operation of different strategies could promote changes in the following:
- Roadway volumes (throughput);
- Roadway speeds;
- The duration of incidents;
- Traveler route choice;
- Traveler mode choice;
- Number of trips made by travelers;
- Number of crashes;
- Severity of crashes; or
- Day-to-day agency procedures, among many other possible changes.
These changes represent the direct impacts of the strategies; however, these changes do not necessarily represent the benefits of the strategy. For example, a change in travel mode promoted by a strategy does not necessarily represent a benefit. The benefit of the change in mode is estimated by measuring the effect that the change in mode has on other more quantifiable metrics, which can be more appropriately valued in monetized terms. Therefore, the impact of the change in mode is measured by the resulting change in several MOEs,such as vehicle operating costs, fuel use, emissions, crash risk exposure, and other measures that may result.
In benefit/cost analysis, the direct and measureable impacts of strategies are often important in that they are used as a means of estimating changes to various MOEs; however, within the benefit valuation framework of the analysis, the impacts are not valued, but are merely an input to the calculations. The sections below provide additional discussion of various MOEs that may be used in assessing TSM&O strategies in a benefit/cost analysis. These are segmented according to:
- Traditionally recognized MOEs;
- Emerging MOEs; and
- Hard-to-quantify MOEs.
Traditionally Recognized MOEs
There are a number of MOEs that are often used in assessing TSM&O strategies. In large part, these MOEs are derived from and consistent with many of the measures used in assessing more traditional capacity related transportation improvements. This is fortunate since the consistency in many of these measures promotes and allows the comparison of the relative benefits across different types of strategies. Additionally, the long-term use of many of these measures has often resulted in a greater consensus regarding the established valuations that may be applied to monetize the benefit.
The most typically applied benefit/cost MOEs include:
- User travel time savings;
- User vehicle operating costs;
- Crashes; and
- Emissions.
These typically used MOEs are summarized in the discussions below. Chapter 4 of this Desk Reference identifies various existing tools and analysis methods that may be used to estimate these measures; and additionally, Chapter 5 of this Desk Reference provides specific guidance in estimating these MOEs using the various analysis tools and methods.
User Travel Time Savings
(Note: The use of consumer surplus and travel time reliability in the measure of travel time-related benefits are discussed in a subsequent section on “Emerging Measures of Effectiveness”)
User travel time is the most often used MOE for a wide range of transportation improvements, including both TSM&O and more traditional capacity-enhancing projects. At its simplest level, user travel time represents the net change in the sum of all person hours of travel (PHT), resulting from implementation of the strategy within the defined geographic scope of the analysis. The travel time, as measured by PHT, may be differentiated by:
- In-vehicle travel time – PHT incurred in the mode of choice of the individual; and
- Out-of-vehicle travel time – PHT necessary to access the mode of choice.
This differentiation is more often used in analyses involving transit modes and is used to represent the time necessary to walk to the transit stop, wait for the transit vehicle, and wait at any transfer locations, but may also be used with auto modes to represent the time necessary to access (walk to) the vehicle in locations where parking is not immediately adjacent to the desired destination.
Depending on the analysis, the PHT may be estimated separately for different modes. This may be done simply to provide an estimate of benefits by mode, or because different benefit valuations will be applied to travel time incurred in different modes. Most typically, truck travel time is differentiated from auto and transit travel time due to the higher costs associated with truck travel time (costs of the driver salary and the carrying costs of the vehicle cargo).
Figure 3-1 shows an example analysis of the daily and annual incremental change in user travel time between a baseline and alternative scenario, where the measure of user travel time was broken out separately for auto, truck, and transit modes. Further, the transit PHTs were also broken out by in-vehicle and out-of-vehicle travel time.
Figure 3-1. Example Display of User Travel Time MOE
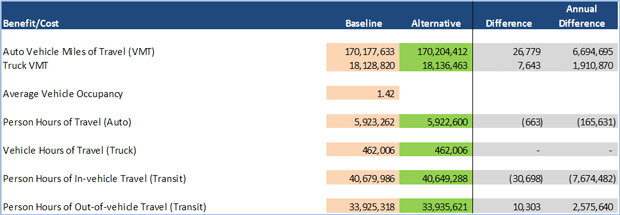
Source: San Francisco Bay Area MTC, 2035 Long-Range Transportation Plan Project Performance Analysis, 2011.
Also depending on the benefit valuation scheme to be applied and the rigor of the analysis, the net change in PHT may be segmented by trip purpose. The most typical categorization includes:
- On-the-clock travel – Represents those business people traveling to a meeting during work hours (e.g., a plumber traveling to the next work site); (Generally, all truck mode travel time is considered “on-the-clock” travel time, unless valued separately.)
- Commuter travel – Represents those individuals traveling between their homes and their business locations; and
- Nonwork travel – Represents individuals making trips for shopping, school, recreation, or other purposes.
The reason for differentiating these trip purposes is to apply a different value of travel time based on the nature of the trip. Travel time incurred during on-the-clock trip purposes may often be valued at a higher rate than nonwork travel in an analysis due to more the greater direct costs incurred in any delay in this type of travel.
Although the user travel time measure is commonly used in benefit/cost analysis, there are limitations to using the measure in average, recurring travel time to assess TSM&O strategies. Many TSM&O strategies are targeted specifically at reducing nonrecurring travel time – due to incidents, inclement weather, excessive demand (special events), and construction activity – therefore, only using measurement of recurring travel time will result in the severe understatement of benefits due to TSM&O strategies. In recognition of this limitation of using average, recurring travel time, the measure of nonrecurring travel time or travel time reliability (variability) has become an important emerging trend in benefit/cost analysis. The travel time reliability measure is discussed in a subsequent section on emerging MOEs.
Source of Measure Estimation in the Analysis. The method of estimating the net change in user travel time in the analysis can range from simple, nearly back-of-envelope type estimations of change to complex analysis involving detailed data and models to calculate the expected changes in transportation system demand and performance. The method and tool used to estimate this MOE should be appropriately scaled to the needed rigor of the analysis. Chapter 4 and the TOPS-BC tool both provide additional guidance on selecting and applying appropriate analysis tools and methods.
At the simplest level, the change in user travel time may be estimated by applying a factor representing the anticipated impact of the strategy to a baseline measure (representing either a current or a future forecast of travel times without the strategy). Many of the sketch-planning tools described in Chapter 4 provide a slightly advanced application of this methodology.
If greater confidence in the accuracy of the analysis results is called for by the needs of the analysis, the estimates of the net change in user travel time may be obtained from more advanced modeling techniques, such as travel demand models or simulation models. These models are also often capable of estimating the change in travel time by mode and/or by trip purpose, if the analysis requires that level of categorization. For detailed evaluation of exiting strategies, archived data sources may also be used to generate the input data (with improvement and without improvement) needed to estimate the net travel time change. Chapter 4 of this Desk Reference provides additional information on the available tools and methods for analyzing user travel time. Chapter 5 provides additional guidance on applying these techniques.
User Vehicle Operating Costs
The use of changes in net vehicle operating costs in benefit/cost analysis of TSM&O strategies is common practice. This MOE is typically broken out into fuel use and nonfuel use categories; although in simple analysis, they may be combined as a single measure. Nonfuel costs typically include measures of maintenance, insurance, and depreciation costs, but do not include vehicle registration and taxes, as these are often considered transfers in benefit/cost analysis.
Similar to the use of user travel time, measures of vehicle operating costs are also often used in assessments of non-TSM&O strategies, increasing the comparability of benefit/cost results across a variety of project types.
Source of Measure Estimation in the Analysis. Estimation of vehicle operating costs is usually relatively easy to estimate, and is often based on simple valuations applied directly to vehicle miles of travel (VMT). For simple analysis, a static rate of average fuel use (gallons per VMT) is applied to any net change in VMT to estimate the net change in fuel use. A benefit value (cost per gallon of fuel exclusive of fuel taxes) is then applied to the change in the number of gallons of fuel consumed. Note that since estimated fuel use rates can differ substantially based upon the year of analysis (future year vehicle fleets are anticipated to have greatly improved fuel use rates), practitioners are encouraged to use rates appropriate to the year of analysis. The U.S. Environmental Protection Agency (EPA) provides data on the past and future anticipated vehicle fleet fuel usage rates. (Fuel Economy)
Although an average fuel use rate may be applied for simple analyses, a fuel use rate sensitive to actual travel speeds may be appropriate for more rigorous analyses, since fuel use is directly associated with travel speeds. For these analyses, a look-up function needs to be applied to link the appropriate fuel use rate to a facility based on the average speed noted for the roadway. The appropriate fuel use rate (in gallons per VMT) may then be applied to the roadway VMT. Different rates are applied to freeway and arterial facilities for any given speed range.
In these analyses, any net change in total VMT or roadway speed attributable to the TSM&O strategy will affect a change in fuel use. Fuel use rates supplied by the U.S. EPA and other sources are applied according to speed and vehicle type (e.g., auto, gas truck, diesel truck) to obtain total fuel use. Table 3-1 presents a sample of year 2010 fuel use rates as specified by U.S. EPA.
Table 3-1 Sample EPA Fuel Use Rates by Average Speed for Year 2010 (in Gallons per VMT)
Facility type |
Speed |
Auto |
Truck Gas |
Truck Diesel |
Freeway |
0 |
0.540 |
0.650 |
0.450 |
5 |
0.182 |
0.310 |
0.696 |
10 |
0.123 |
0.181 |
0.489 |
15 |
0.089 |
0.135 |
0.297 |
20 |
0.068 |
0.118 |
0.185 |
25 |
0.054 |
0.120 |
0.131 |
30 |
0.044 |
0.133 |
0.110 |
35 |
0.037 |
0.156 |
0.112 |
40 |
0.034 |
0.185 |
0.122 |
45 |
0.033 |
0.223 |
0.136 |
50 |
0.033 |
0.264 |
0.153 |
55 |
0.034 |
0.310 |
0.170 |
60 |
0.037 |
0.374 |
0.187 |
65 |
0.043 |
0.439 |
0.204 |
70 |
0.052 |
0.511 |
0.221 |
Arterial |
5 |
0.144 |
0.275 |
0.383 |
10 |
0.091 |
0.174 |
0.241 |
15 |
0.073 |
0.140 |
0.194 |
20 |
0.064 |
0.123 |
0.171 |
25 |
0.059 |
0.113 |
0.157 |
30 |
0.056 |
0.106 |
0.147 |
35 |
0.053 |
0.101 |
0.140 |
40 |
0.051 |
0.097 |
0.135 |
Source: Fuel Economy.
For nonfuel operating costs, an established value in these costs per VMT are directly applied to the change in VMT to estimate the benefit value. Similar to fuel use rates, the per VMT value may be sensitive to the forecast year of analysis; however, the valuation is usually not sensitive to differences in operating conditions (travel speeds, etc.).
Crashes
The assessment of safety impacts, particularly the risk of exposure to crashes, is typically a critical part of any transportation benefit/cost analysis. Many TSM&O strategies have proven to have significant benefits in the reduction of the number and/or severity of vehicle crashes. Strategies that serve to smooth the flow of traffic at difficult merge points, such as ramp metering systems, have been observed to directly reduce the risk of crash exposure at those locations. Meanwhile, applications such as traffic incident management that serve to improve the emergency response to crashes have been demonstrated to reduce the severity of crashes by quickening the response times and better ensuring the appropriate emergency responder assets are directed to the scene when crashes occur.
The benefit valuations typically applied to a reduction in the number of crashes are often substantial, particularly in the case of fatality and injury crashes, ranging into multiple millions of dollars. Often, these valuations are based on a two-tiered structure to fully capture the benefits of a reduction in the number or severity of a crash. Typical crash reduction benefit valuations are based on a combination of the following valuation methods:
- Actual costs – This valuation methodology is intended to capture the actual accountable costs of the crash. For example, in the case of a fatality crash, these costs would include the costs of medical treatment of the victims, the loss of the victim’s wages for the family, and any property damage.
- Cost to avoid – Most crash valuations additionally include a measure of the additional cost to avoid; or in other words, an estimate of the value that individuals would likely pay to avoid being involved in a crash of this severity. This valuation additionally accounts for the potential pain and suffering experienced by the crash victim(s) and their families.
Source of Measure Estimation in the Analysis. To estimate the change in the number of crashes attributable to a TSM&O strategies, crash rates (typically expressed as crash occurrence per million VMT) are applied to the change in VMT in the network or for a particular facility type (freeways versus arterials). Different crash rates are also usually applied for different severities of crashes, most generally defined as:
- Fatality crash;
- Injury crash; and
- Property damage only crash.
However, many different agencies use different categorization schemes for crashes; often breaking injury crashes into more discrete severity categories (minor injury versus severe injuries). Many agencies also maintain their own crash rates that are based on local crash records, and are typically the best source of rates for use in any TSM&O benefit/cost analysis since they are locally derived and consistent with analysis performed for other non-TSM&O strategies.
At the simplest analysis level, a single static rate (for each crash severity) may be applied to the total change in VMT for all vehicle categories estimated from the application of the TSM&O strategies. For more rigorous analysis, more segmented and dynamic rates may be researched and applied. These enhanced rates may be categorized or sensitive to:
- Vehicle types (autos versus trucks);
- Facility types;
- Roadway configuration and geometrics;
- Existing roadway safety installations;
- Facility speeds;
- Facility congestion levels (most often facility volume/capacity ratio); and/or
- Even applied to individual links based on actual historical crash occurrence.
The level of sensitivity of the crash rates to be applied in any given analysis is typically based on the following factors:
- The locally prescribed crash analysis rate;
- The need to maintain consistency with other local analysis;
- The analysis rigor (level of confidence required in the analysis results);
- The type of TSM&O strategy being analyzed (e.g., some TSM&O strategies may be anticipated to have more direct crash savings benefits, and thus may require additional analysis rigor to quantify these benefits);
- Availability of enhanced rates; and
- Availability of appropriately structured input data (e.g., VMT by facility type and vehicle type) to support the crash analysis structure.
Beyond the analysis of crash occurrence based on estimated changes in VMT, many TSM&O strategies additionally may influence the actual crash rate that should be applied. For example, ramp metering applications serve to smooth traffic merging at the confluence of traffic at the end of the on-ramp lane. This smoothing of the merge has been observed to result in a meaningful decrease in the crash rate (particularly for sideswipe, rear end, and run-off-road accident types that are common in merge areas). Previous ramp meter applications deployed nationwide have been observed to reduce crashes in the merge area by approximately 7 to 25 percent. This crash rate reduction is in addition to any change in the number of crashes resulting from changes in the underlying VMT. Similarly, strategies such as speed harmonization applications are intended to smooth the flow of traffic and reduce the amount of stop-and-go movements. These applications would likely reduce the risk of crashes without any change in the underlying VMT at that location.
In these situations, where the TSM&O strategy has a direct linkage to reduced crashes, the crash rate that is applied at locations influenced by the TSM&O strategy should be reduced prior to application to the VMT observed for the applicable facility. Using the ramp metering example above, the applicable crash rate would reasonably be reduced by 7 to 25 percent prior to application to the VMT estimated in the merge area following the application of ramp metering.
Emissions
Emissions are another commonly used MOE for TSM&O strategies, as well as traditional infrastructure projects. The inclusion of emissions estimates in the benefit/cost analysis is particularly important when projects are being prioritized in competition for several types of funding (e.g., Congestion Mitigation and Air Quality (CMAQ) funds); or the project is being considered in an air quality nonattainment area.
Emissions categories considered in benefit/cost analysis most often include one or more of the following emissions categories, and typically include those emissions categories of most concern in the local region:
- Hydrocarbons (HC)/Reactive Organic Gases (ROG);
- Nitrous Oxide (NOx);
- Carbon Monoxide (CO);
- Carbon Dioxide (CO2);
- Particulate Matter (PM10) or Fine Particulate Matter (PM2.5); and
- Sulfur Dioxide (SO2).
Emissions may represent one of the most complex to estimate MOEs used in many B/C analyses. This is due to the many variables that determine the appropriate emission rates. Most emissions estimations are based on an application of an emissions rate on a per VMT basis. Depending on the emissions category, the appropriate emissions rate to apply may be sensitive to numerous factors, including the following:
- Year of the analysis – Future year vehicle fleets are anticipated to produce fewer emissions in many categories;
- The mix of gasoline and diesel vehicles in the regional fleet;
- Vehicle speeds;
- The number of cold starts;
- The mix of vehicles in the regional vehicle mix (e.g., autos, light trucks, medium-duty trucks, heavy-duty trucks, etc.); and
- Regional weather patterns/climate and other considerations.
Given these many variables impacting emissions rate, the rates for most analysis need to be obtained from existing emissions analysis tools specifically configured to the individual region, or derived from previously conducted regional analysis. Therefore, caution should be applied in using rates derived from other regions or based on averages between different regions.
Source of Measure Estimation in the Analysis. Many urban areas, particularly those in nonattainment areas, maintain robust emissions estimation and analysis models and tools. These are most typically based on the U.S. EPA tools, including MOBILE or MOVES, for most of the country. In California, emissions analysis is based on tools and guidance developed by the California Air Resources Board (CARB). These customized and configured tools are typically the best source of analysis of emissions impacts, when available. These tools and analyses are typically linked to analysis conducted in the regional travel demand model; and in many cases, the emissions analysis is performed as a post-processing of the travel model data outputs, or may also be performed as an iterative feedback loop with the travel demand model.
In areas when an appropriate regional air quality model is unavailable or situations where running the full analysis model is impractical given the time and budget resources available for the analysis, more simplified analysis may be necessary. Simplified emissions analysis may be conducted using emissions rate tables derived from the regional emissions analysis process combined with a look-up function to ascertain the appropriate rate (based on vehicle class and speed) to apply. Several sketch-planning and post-processing analysis tool (discussed in more detail in Chapter 4) use this simplified approach for their own internal emissions analysis. Practitioners using this simplified method need to use caution in selecting rate tables that are appropriate for the region and time periods being analyzed.
Emerging MOEs
In recent years, several new MOEs have been introduced into many B/C analyses, including measures of travel time reliability (variability), consumer surplus, and measures of global climate change. These measures can often provide more justification for TSM&O projects as these measures, particularly travel time variability, are precisely the focus of many Operations deployments.
Travel Time Reliability
Practitioners and researchers have increasingly been assessing the importance of travel time reliability when evaluating transportation projects. Historically, average travel time was the primary measure most often used for B/C analysis of transportation projects. However, use of this average measure often only captures the change in recurring travel time, due to the nature of the measure itself and the methods that were often used to assess the measure. As shown in Figure 3-2, use of the average travel time may not realistically represent actual traveler’s experiences.
Figure 3-2. Use of Recurring Travel Time Measurement versus Actual Traveler Experience
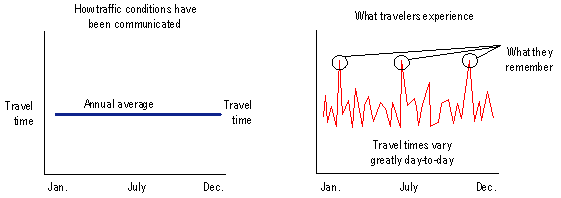
Source: Freight Performance Measurement: Travel Time in Freight-Significant Corridors.
More recently, significant research and analysis have been performed on the effects of nonrecurring travel time delay – travel time delays caused by factors, such as incidents, special events, weather, construction work zones, and poorly timed traffic signal systems, among other causes. Research completed as part of the Transportation Research Board’s (TRB) Strategic Highway Research Program (SHRP 2) and other numerous Federal and state efforts have shown the amount of delay caused by nonrecurring congestion is substantial; and leaving this measure out of transportation B/C analysis risks severely understating the potential benefits of many improvements. Figure 3-3 presents a breakdown of the typical causes of congestion, as estimated from national sources; and shows that nonrecurring sources of congestion account for more than one-half of the total delay in a typical urban network.
Figure 3-3. National Summary of the Sources of Congestion
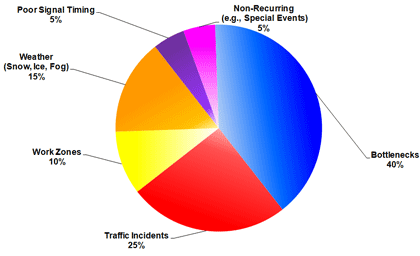
Source: Cambridge Systematics, Inc., and Texas Transportation Institute, Traffic Congestion and Reliability: Linking Solutions to Problems, July 19, 2004.
Travel time reliability measurement seeks to quantify the variability in travel times caused by nonrecurring, as well as recurring, congestion sources in order to better estimate the full distribution of travel times experienced by the system users. A number of performance measures and indices have been developed to help quantify these impacts, as shown in Table 3-2. (Note: Although many of these performance measures are useful in assessing system reliability, they are not appropriate as MOEs to be used in B/C analysis since they provide relative comparisons of reliability levels that are not immediately able to be valued (monetized).) Many of these measures are based on an analysis of the distribution of travel times for a particular segment or facility.
Table 3-2 Reliability Performance Measures
Reliability Performance Metric |
Definition |
Units |
Planning-Time Index |
- 95th percentile Travel Time Index (95th percentile travel-time divided by the free-flow travel time), normalized by the average travel time
- The difference between the 95th percentile travel time and the median travel time, normalized by the median travel time
|
None |
Buffer Index (BI) |
- The difference between the 95th percentile travel time and the average travel time, normalized by the average travel-time
- The difference between the 95th percentile travel time and the median travel time, normalized by the median travel-time
|
Percent |
Failure/On-Time Measures |
- Percent of trips with travel-times less than 1.1 * Median Travel Time or 1.25 * Median Travel Time
- Percent of trips with space mean speed less than 50 mph, 45 mph, or 30 mph
|
Percent |
80th Percentile Travel-Time Index |
- 80th percentile travel time divided by the free-flow travel time
|
None |
Skew Statistic |
- The ratio of (90th percentile travel time minus the median) divided by (the median minus the 10th percentile)
|
None |
Misery Index (Modified) |
- The average of the highest five percent of travel times divided by the free-flow travel time
|
None |
Source: SHRP 2 Project L03 Final Report: Analytical Procedures for Determining the Impacts of Reliability Mitigation Strategies.
In addition to the research work completed on improved measures and analytical methods for assessing travel time reliability in a network, many parallel efforts have been undertaken to demonstrate the impact that many different types of transportation strategies have in improving travel time reliability. Both traditional types of capacity expansion projects and TSM&O strategies have been shown to improve reliability. Although most typically focused on recurring congestion issues, traditional capacity increasing projects may improve reliability since roadways and transit facilities with more available capacity are more easily able to handle unusual spikes in demand or weather the impacts of an incident. TSM&O strategies are often more directly targeted at the underlying causes of nonrecurring congestion – incidents, weather, construction work zones, special events, and poor operations – and can have substantial success in mitigating the delay caused by these sources. Therefore, it is important that travel time reliability be considered for traditional capacity projects; however, it is often even more critical that this measure be considered for B/C analysis of TSM&O strategies, since a much higher proportion of the anticipated overall benefit is expected to be derived from these strategies’ impacts on reducing nonrecurring congestion. (SHRP 2 L03 Final Report: Analytical Procedures for Determining the Impacts of Reliability Mitigation Strategies, 2010.)
Source of Measure Estimation in the Analysis. To meet the need to be able to better consider valuation of travel time reliability benefits within a benefit/cost analysis structure, many new and enhanced methods for quantifying this measure have been proposed, developed, and tested. However, as much of this research is still evolving, there are frequently limitations in applying many of the techniques. Additional research is ongoing to refine and enhance the approaches; some of which are detailed below.
Measurement of standard deviation. For existing deployments where sufficient “before and after” data is available, the impact on travel time reliability may be estimated by comparing the distributions of travel time prior to application of the strategy (the “before” or “without” scenario) with the distribution following the application (the “after” or “with” scenario). The net change in the standard deviation in minutes of travel time may be calculated from the distributions and multiplied with the number of facility users to estimate a temporal measure of travel time reliability savings. A value may be applied to this measure to monetize the benefit for use in the B/C analysis. This type of analysis assumes, however, that an evaluation of an existing deployment is being performed – the method is not as useful as a predictive tool – and that sufficient data is available to support the estimation of the travel time distributions. (Modeling of multiple scenarios representing varying traffic conditions may be used to make this analysis methodology more applicable for predictive use. See Section 5.0 for a discussion of the required analysis structure.) Data required to identify the travel time distributions may be obtained from long-term data archive systems, multiple manual data collection/monitoring activities, or modeling efforts using multiple scenarios representing varying expected traffic conditions (see Chapter 5 for an expanded discussion of structuring modeling analysis to consider impacts during varying traffic conditions). Regardless of the data source, the analyst needs to ensure that the dataset used to estimate the distribution of travel times is sufficiently robust to fully capture travel times during a representative sampling of travel time conditions. This may require six months to multiple years’ worth of constant data in some situations, where a high level of confidence in analysis results is required.
Measurement of total recurring and nonrecurring delay. The development of the SHRP 2 L03 Report, Analytical Procedures for Determining the Impacts of Reliability Mitigation Strategies, resulted in the refinement of an approach to estimate total delay (combining both recurring and nonrecurring delay), based on the mean recurring travel time and assuming a normal distribution of travel times. The developed analysis method and equation are intended for application in “data poor” situations, where the robustness of either the archived historic traffic data or the modeled predicted traffic data was insufficient to provide meaningful analysis of nonrecurring congestion delay. The function below, which is intended to be applied to freeway facilities, was estimated by statistically examining the relationship between the total mean travel time delay and the mean recurring travel time estimate (as based on long-term data collected and analyzed from multiple regions throughout the nation); and allows an adjustment of the mean recurring travel time estimate to include the addition of an estimate of nonrecurring travel time. (SHRP 2 L03 Final Report, Analytical Procedures for Determining the Impacts of Reliability Mitigation Strategies, 2010.)
MeanTT = 1.0274 * RecurringMeanTT1.2204
The above equation provides a reasonable approximation of the relationship between overall congestion and recurring congestion. The method does produce a measure of total recurring and nonrecurring delay that may be summed for all facilities and users, compared for a baseline and alternative scenario to identify a net change in the measure, and monetized to provide an estimate of travel time reliability in the B/C analysis. However, its application in B/C analysis for TSM&O strategies is limited, primarily due to the inability to effectively break out recurring travel time from nonrecurring travel time (travel time reliability), and the difficulty in assessing the specific impact that particular TSM&O strategies may have on reducing specific causes of nonrecurring congestion since any change in total mean travel time is only based on changes in mean recurring travel time.
Additional work is currently underway as part of a subsequent SHRP 2 project to allow for more effective breakout of the nonrecurring congestion by source; and to develop additional analysis methods to estimate the impacts of various TSM&O strategies on the source causes of congestion, thus allowing broader application of this methodology. (SHRP 2 L05 Draft Interim Report, Incorporating Reliability in the Transportation Planning Process, 2011.)
Incident related delay. As shown previously in Figure 3-3, traffic incidents account for approximately 25 percent of all congestion delay and the most substantial proportion of nonrecurring (nonbottleneck related) delay sources in most urban areas. As a result, several analysis methods have been specifically developed to estimate incident-related delay.
The most prevalent example of these methods is the analysis originally developed and implemented as part of the analysis capabilities of the FHWA’s IDAS sketch-planning tool. (IDAS.) The IDAS incident delay analysis involves the use of look-up rate tables containing estimates of the amount of incident-related delay likely to be experienced for a facility on a per VMT basis. These rates were predicted based upon long-term monitoring and analysis of annual incident delay experience on a number of national freeway corridors. These rates are sensitive to several key input factors, including the following:
- The number of facility lanes – The incident-related delay rate for freeways with a greater number of lanes is less than for facilities with fewer lanes, assuming other factors are also equal. This is due to the more substantial blockage of capacity caused by similar incidents on roadways with fewer lanes. For example, identical incidents blocking a single lane would reduce capacity by one-half of the available lanes on a two-lane facility, but only reduce capacity by one-quarter of the available lanes on a four-lane facility.
- The facility volume-to-capacity (V/C) ratio – The level of base congestion (prior to the occurrence of the incident) is represented by the V/C ratio. Facilities with a higher V/C ratio will have higher incident-related rates associated with them, as it would be expected that incidents on these more congested facilities would cause a quicker breakdown in conditions and a longer time required to allow the incident-related queue to dissipate once the incident was cleared.
Different rates are also estimated based on the length of the analysis period (one-hour, two-hour, three-hour, four-hour, or daily). Table 3-3 presents an example of the travel time reliability rates used in IDAS. These tables may be employed using a look-up function in an analysis to identify the appropriate rate to the VMT estimated for the facility. This estimation would be performed for the baseline scenario (without the improvement) and for an alternative scenario (with the improvement). The amount of incident-related delay would be expected to change if there are any changes in roadway volumes, capacities, or number of lanes as a result of the improvement in the alternative scenario as compared to the baseline scenario.
Table 3-3 Sample IDAS-Derived Travel Time Reliability – Rates for One-Hour Peak
(Vehicle Hours of Incident Delay per Vehicle Mile)
Volume/1-Hour Level of Service Capacity |
Number of Lanes |
2 |
3 |
4+ |
0.05 |
3.44E-08 |
1.44E-09 |
4.39E-12 |
0.1 |
5.24E-07 |
4.63E-08 |
5.82E-10 |
0.15 |
2.58E-06 |
3.53E-07 |
1.01E-08 |
0.2 |
7.99E-06 |
1.49E-06 |
7.71E-08 |
0.25 |
1.92E-05 |
4.57E-06 |
3.72E-07 |
0.3 |
3.93E-05 |
1.14E-05 |
1.34E-06 |
0.35 |
7.20E-05 |
2.46E-05 |
3.99E-06 |
0.4 |
0.000122 |
4.81E-05 |
1.02E-05 |
0.45 |
0.000193 |
8.68E-05 |
2.34E-05 |
0.5 |
0.000293 |
0.000147 |
4.93E-05 |
0.55 |
0.000426 |
0.000237 |
9.65E-05 |
0.6 |
0.0006 |
0.000367 |
0.000178 |
0.65 |
0.000825 |
0.000548 |
0.000313 |
0.7 |
0.001117 |
0.000798 |
0.000528 |
0.75 |
0.001511 |
0.001142 |
0.00086 |
0.8 |
0.002093 |
0.001637 |
0.00136 |
0.85 |
0.003092 |
0.002438 |
0.002115 |
0.9 |
0.005095 |
0.004008 |
0.003348 |
0.95 |
0.009547 |
0.007712 |
0.005922 |
1 |
0.01986 |
0.01744 |
0.01368 |
Source: IDAS User’s Manual – Appendix A, 2008.
In addition to changes in incident-related delay forecast due to predicted changes in volume, capacity, or number of lanes, some TSM&O strategies have been observed to lessen the frequency of incidents or reduce their duration. Strategies such as traffic incident management systems have been observed to reduce incident-related delay by up to 40 percent due to the improved and faster response provided by the enhanced incident detection, verification, and coordinated response. (IDAS.) In this analysis situation, the hours of incident-related delay for the facility would first be calculated for the baseline and the alternative using the look-up analysis described in the paragraph above. Then, the resulting estimation of hours of incident-related delay for the alternative scenario would be further reduced by 40 percent (or another factor more appropriate to the local conditions) prior to comparison with the baseline and valuation in the B/C analysis. Further description of how this analysis may be performed is provided in Chapter 5.
The methodology used in IDAS for estimating incident-related delay has been adopted for use in the TOPS-BC tool that supports this Desk Reference. A description of how this analysis is applied is described in the TOPS-BC User’s Manual.
Induced Travel/Consumer Surplus
The concept of consumer surplus has increasingly been applied in many recent B/C analyses of transportation projects. Consumer surplus is an economic concept intended to enhance the analysis of travel time and costs. It is based on the principle of weighing the willingness of individuals to pay (in time and money) for a particular trip with the actual cost of the trip, and is capable of more accurately assessing the benefits of any induced travel resulting from the improvement.
Each user of the transportation facility is willing to pay a certain price (in terms of time, accident risk, vehicle operating costs, tolls, etc.) to travel on that facility. This amount will differ from user to user. However, every user typically incurs the same travel time costs. The difference between what a group of users is willing to pay in terms of travel costs (travel time, etc.) and what they actually pay is called consumer surplus. In a benefit cost analysis, we are concerned with the change in consumer surplus attributable to a transportation improvement.
Measurement of consumer surplus is most often used in the assessment of travel time savings in place of simple measures of the total change in facility travel time observed between the baseline and alternative scenarios. An example of why consumer surplus may be a more accurate picture of travel time benefits, as opposed to using an approach that only considers the total change in travel time, is provided here. A section of roadway in the baseline scenario carries 6,000 vehicles during a peak period and maintains an average travel time of 20 minutes, resulting in 2,000 hours of vehicle travel time. In an alternative scenario, the segment travel time is reduced to 18 minutes as a result of a deployment; however, this improvement in travel time promotes an additional 800 travelers, who previously chose to forego their trips, to now travel, resulting in 6,800 trips on the facility. This would result in a total of 2,040 hours of travel time in the alternative scenario. If only total travel time was used to estimate the benefits of the improvement scenario, the analysis would indicate that the transportation improvement resulted in a disbenefit, since there would be 40 more hours of travel time incurred in the alternative scenario as compared with the baseline scenario.
The estimation of a travel time disbenefit in the above example is counterintuitive since all the existing travelers incur less travel time to traverse the segment; and the new induced travelers must also be benefiting from using the facility, or they would not have chosen to change their behavior. When consumer surplus is estimated, the impacts of new, induced travel are treated separately to account for this effect. Typically, a linear relationship is assumed in the elasticity of individuals’ sensitivity to travel in response to costs, therefore, allowing new traveler benefits to be estimated as one-half (50 percent) of the benefits of existing users. The following two formulas would be used to estimate consumer surplus benefits for existing and new users of a transportation facility:
Where:
V1 = Baseline volume
V2 = Alternative volume
P1 = Baseline price (travel time)
P2 = Alternative price (travel time)
Existing users = V1 * (P1 – P2)
New users = 0.5(V2 – V1)(P1 – P2)
Using the hypothetical example provided above, this consumer surplus would be calculated as follows:
Consumer Surplus Existing Users = 6,000 * (20 min – 18min) = 200 hours
Consumer Surplus New Users = 0.5 * (6,800 – 6,000) * (20 min – 18 min) = 13.3 hours
The sum of these two values represents the estimated change in consumer surplus due to the transportation improvement, or:
Total Consumer Surplus = 200 hours + 13.3 hours = 213.3 hours saved
Thus, the use of consumer surplus in place of standard total travel time benefit in this analysis results in a more accurate depiction of the improvement as having positive travel time benefits. Since the measurement of consumer surplus is closely linked with induced travel, it is most often used in analyses that are anticipated to result in significant numbers of induced trips being made. (Although the discussion in this section focuses on the effects of induced trips, consumer surplus can be equally applied to capture the disbenefits of individuals deciding to forego travel in response to an increase in travel time or the cost of travel.) If only a small number or no new trips are expected to be made as a result of the improvements being analyzed, there is little advantage in using consumer surplus, since the estimation of benefits will not vary significantly from the benefits estimated using total travel time.
Note: “New” trips in the analysis are only intended to represent those travelers who previously did not make a trip, but were induced to travel by the lowered cost of making the trip. Increased volumes of trips resulting from route changes from parallel roadways or mode shifts from other forms of transportation should not be treated as induced travel in this analysis method.
Difficult to Quantify MOEs
Beyond the more traditional and emerging MOEs discussed above, a number of additional potential benefits of TSM&O strategies have been identified and studied. Meaningful estimation of many of these MOEs within the confines of a B/C analysis framework has often proven difficult, however. Barriers to developing accurate quantification of these barriers often include:
- Few existing estimation/analysis methods;
- Little established consensus on the appropriate valuation to place on the change estimated for the MOE;
- Potential overlap with existing measures (increasing the likelihood of double-counting of benefits);
- Quantification of the measures is not supported by generally used transportation analysis tools; and
- Little empirical evidence establishing a link between TSM&O strategies and quantifiable changes in the measures.
Several examples of these difficult to quantify MOEs include:
- Community livability – Improvements to the desirability of a community to residents due to the more efficient and effective operation of the transportation system. Inclusion of these benefits in analysis of TSM&O strategies is often hampered by the lack of a clear consensus of how TSM&O strategies affect livability in a community, as well as appropriate methods for estimating and valuing this measure.
- Customer satisfaction – Many TSM&O strategies have been shown to have a strong linkage to improving customer (traveler) satisfaction. Surveys and customer comments related to improvements, such as freeway service patrols and traveler information systems, are often highly positive and supportive of these types of improvements; however, there often remain significant barriers to establishing appropriate valuations of these benefits in a B/C analysis.
- Traveler feelings of safety and security – Some TSM&O strategies have been shown to enhance travelers’ feelings regarding the safety and security of travel. For example, surveys and system user comments have revealed that travelers highly appreciate the availability of freeway service patrols (FSP). Even travelers who have never had to use the services first-hand often feel more secure in their travels knowing that they are less likely to be stranded in an uncomfortable or dangerous situation given the presence of the FSP. Likewise, strategies that serve to smooth the flow of traffic and reduce crash risk exposure (e.g., an application of ramp meters at a difficult merge location) may also serve to reduce the anxiety of users traveling through the improvement location. This benefit is typically not captured in the valuation of crash occurrence, mostly due to difficulties in valuing (e.g., assigning a dollar value) to the benefit.
Given the difficulties in quantitatively assessing many of these MOEs, these benefits are more often analyzed using more qualitative assessment external to the B/C analysis. As more information is gathered on these measures and the linkage of TSM&O strategies to these measures, more enhanced quantitative analysis may be possible in the future.
You will need the Adobe Reader to view the PDFs on this page.