Raising Awareness of Artificial Intelligence for Transportation Systems Management and Operations
Chapter 5. Considering Artificial Intelligence Technologies in Transportation Planning, Deployment, and Operations
Artificial intelligence (AI) and machine learning are elements of business intelligence (BI) strategies and technologies, which are used by enterprises for data analysis and information extraction. Traditional problems, functions, or actions that AI techniques can address include reasoning, knowledge representation, planning, learning, natural language processing (NLP) (and understanding), perception, and the ability to move and manipulate objects.98 In each problem area, AI technologies are proving to have significant performance benefits versus other traditional mathematical modeling approaches. For example, the capabilities of digital assistants to understand human speech significantly outperforms the interactive voice response (IVR) technologies used in 511 systems over the past 20 years.
Various types of AI technologies could be used to help infrastructure owner-operators (IOO) for transportation systems management and operations (TSMO). For example, neural networks may help agencies improve incident detection time and identify anomalous activity, such as pedestrians or debris on a freeway; fuzzy logic may simplify the configuration of ramp metering systems; natural language question-and-answering systems may expand the abilities of TSMO staff to quickly obtain information from databases of facts and figures; and unmanned aerial systems (UAS), both on the ground and in the air, will likely augment human workers in a variety of ways. The selection of AI technologies is dependent on the particular problem at hand.
As discussed in chapter 3, the development of AI technologies is currently becoming commercialized. AI development platforms are now available from major software providers such as Google, Amazon, and Microsoft as well as hundreds of other niche and generalized companies, including companies focused solely on the transportation management market. Each of the major technology providers continue to improve the capabilities of their suite of software modules for AI to make the development of applications easier and more accessible to users without deep and extensive expertise in machine learning. At the time of this writing; however, it is still the case that AI applications for TSMO will require extensive involvement from experts in AI, software development, and database management. Procurement of AI applications is currently a complex undertaking, which may range from purchasing a software package from a vendor to developing in-house applications using cooperative University resources for research and development.
In chapter 4, a few examples of existing applications of AI to TSMO problems were presented. These examples illustrate some of the potential for AI in TSMO, particularly in imagery analysis for incident detection and the automation of transportation management centers' (TMC) functions through digital assistants. Aside from the fuzzy logic methods that have been used for ramp metering by Washington State Department of Transportation (DOT) for an extended period, most AI application examples are exploratory and have been recently deployed. Most applications have seen significant performance improvements, although benefit/cost analysis has not been completed and documented.
In this chapter, you will discover issues that should be considered in planning, deployment, and operations of AI technologies for the TMC, and transportation management systems (TMS). This includes the identification of:
- Clear objectives to be addressed with AI.
- Systems and technology that are required for different types of AI applications.
- Changes or enhancements to staffing and organization may be needed for deployment of AI.
- Business processes that may need to change.
- Types of collaborations that can be made with other DOT divisions or other State and local agencies to reduce cost and complexity of deploying AI applications.
This chapter is organized around a subset of the topical areas of the capability maturity model for TSMO:
Each of these subareas will be discussed and checklists of questions and issues will be provided for your use in generating an AI concept of operations.
There are many issues that will be common in any AI program development, including systems engineering, deployment, operations, and maintenance and new capabilities.
Infrastructure owners and operators will need to employ systems engineering of new concepts and applications in considering:
- The deficiencies of current TSMO activities are to be addressed by use of AI systems.
- The improvements gained by using AI systems versus traditional systems.
- The maturity of AI technology to support a specific application.
- The prototype status of AI tools and technologies and consensus on practical "day one" applications.
- The appropriate software systems, databases, and computing resources.
- The integration of new systems and software with legacy systems and software.
- The capabilities of IOOs to adjust processes to appropriately take advantage of new functions.
- The use of AI technologies beyond TSMO and collaborations with other DOT departments or other departments within a State or local agency.
The development, deployment, operations, and maintenance of new AI systems may place significant burdens on agency capabilities in the areas of:
- Policy development and compliance with legal requirements regarding security and privacy.
- Management and integration of AI software that requires highly skilled personnel in short supply.
- Funding constraints.
- Development of new business processes required for AI applications and integration of AI into existing processes.
- Management new types of data and volumes of data with modern tools and technologies.
- Measurement of AI application deployment performance.
Some AI applications may involve coordination and collaboration with local IOOs, metropolitan planning organizations, and coalitions. There is also a likely role for private service providers to collect and process data and implement solutions on behalf of the agency. This implies the need for capabilities in the areas of:
- Developing guidelines and collaborative agreements across regions.
- Establishing new forms of public-private partnerships.
- Considering partnerships and data packaging from third-party commercial providers.
- Developing and retaining specialized staff and knowledgeable management.
- Developing an appropriate organizational framework for coordination and allocation of authority and responsibility.
Systems and Technology
AI applications for TSMO will be based on software, databases, and information technology. Many issues will be needed to be considered in deploying AI. As detailed in chapter 3, most AI applications will include some level of consideration of the Cloud. This includes Cloud data storage, Cloud computing, and leveraging Cloud software services. DOTs range widely in information technology (IT) policies and procedures when considering Cloud applications and technology. Partnering with the IT department early in the project development phase will be an important element of success in developing and deploying AI applications.
The following issues are important to consider in software, databases, and IT:
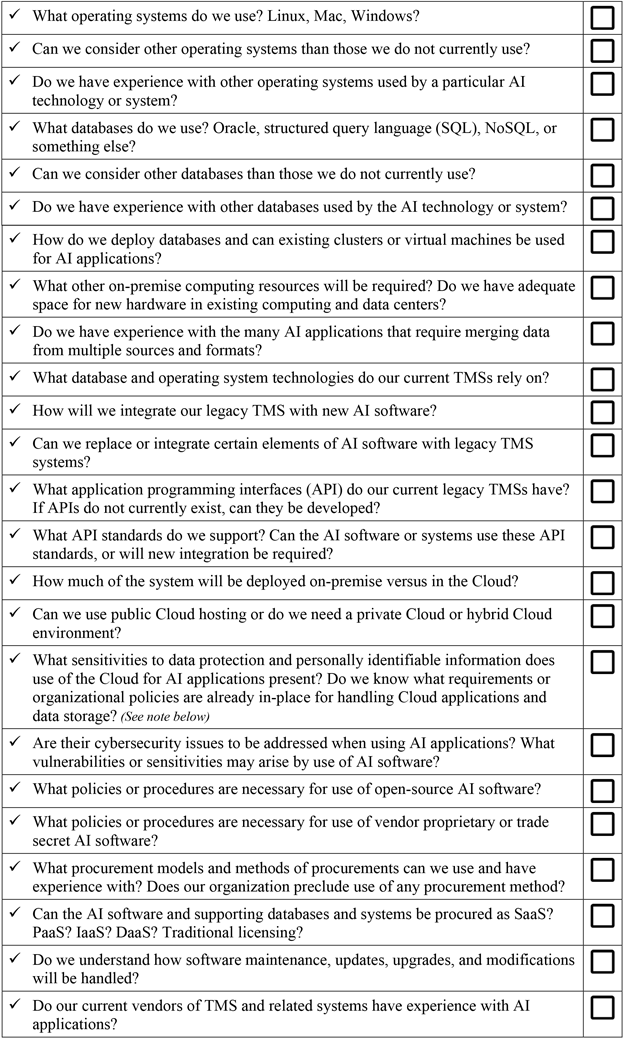
Note: Virginia Information Technologies Agency. "Cloud-based Hosting for IT Solutions Policy." Information Technology Resource Management Policy EA 300-01 (Oct 2018). Accessed December 10, 2019.
Staffing and Organization
Use of AI applications will require technical capabilities that likely exceed existing level of in-house expertise in most IOOs. Similarly, organizational decisions will be required to determine which department(s) or agency staff will be involved in the project and responsible for success.
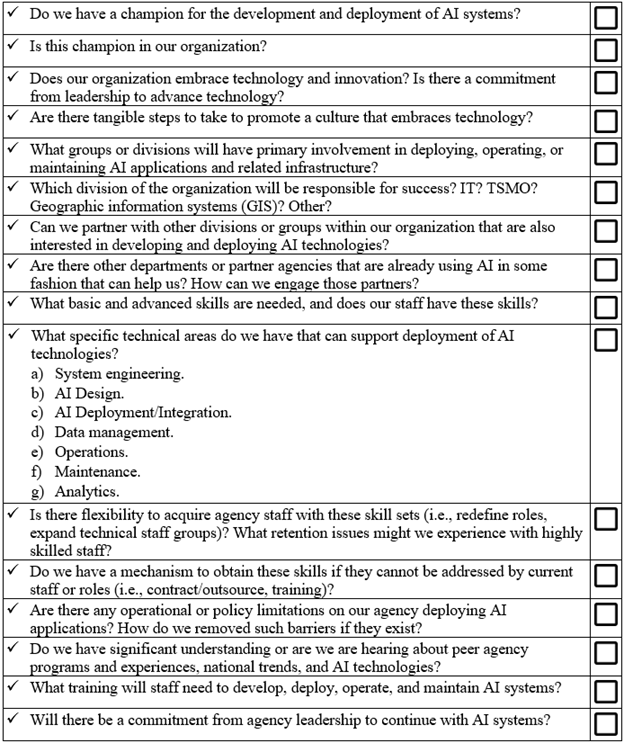
Business Processes
The introduction of AI applications and pilots brings the identification of requirements for planning, scheduling, budgeting, and project development. The introduction of AI technologies will require processes for data collection, exchange, and action. Business processes for AI applications are especially important if the application area is not a TSMO area an agency currently uses, and therefore, has not already initiated efforts to advance business processes through TSMO program planning or related work. Agencies may need new roles at the IOO, such as database and AI technology specialists. For most AI applications, an agency will need an institutionalized approach to cybersecurity and privacy. In the short term, IOOs may have significant technical challenges. In the long term, it is anticipated that these challenges will evolve towards standards and best practices.
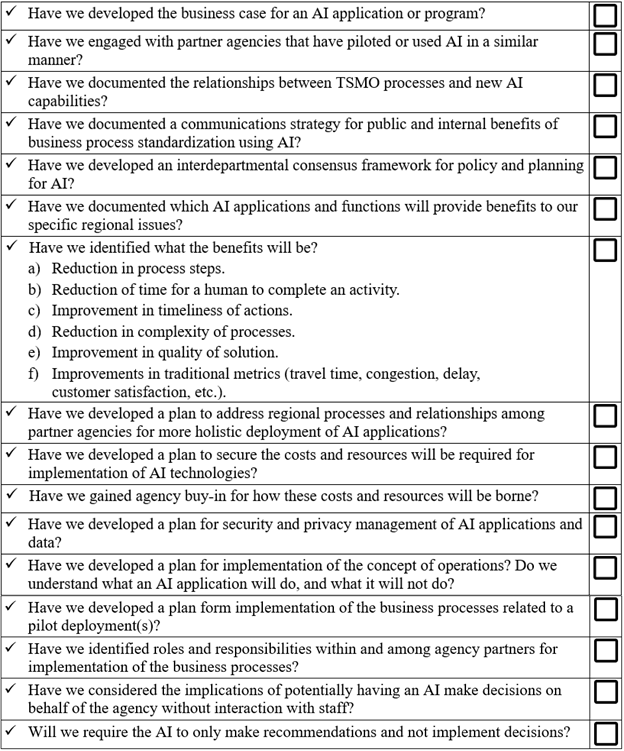
Collaboration with Other Departments and Agencies
AI applications and software/hardware tools are likely applicable to many other organizational groups, departments, and partner agencies. Given the experimental and cutting-edge nature of AI technology, collaboration may be beneficial to share costs, expertise, and responsibilities.
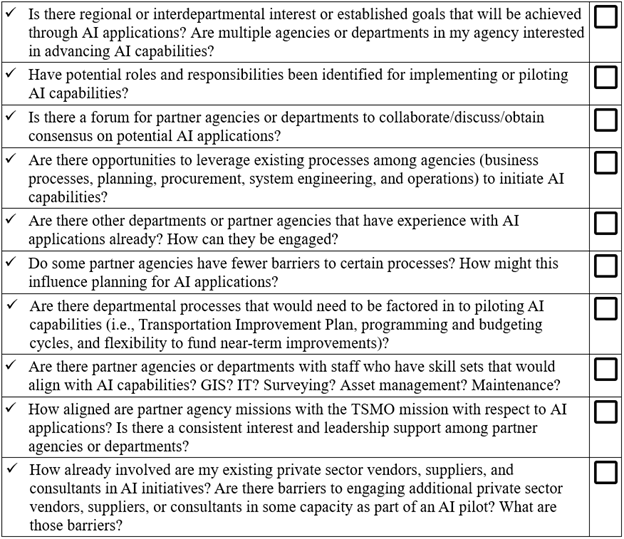
Summary
The previous section listed important questions to answer or address when embarking on the development of AI applications for TSMO. Identifying answers to these basic questions will better prepare transportation agencies for the potential impacts of AI on operational activities, resources, system needs, and decisionmaking. AI technologies are making major headway in commercial software systems, particularly for imagery analysis, pattern recognition and classification, consumer services, such as Google Assistant and Alexa, and automated vehicles on the ground and the air. Leveraging these technologies holds promise for improving TSMO activities.
Determining how to start in AI applications for TSMO will be unique to your organization. As is true with any TSMO activity, the basis for improvement of any activity with AI has three basic components:
- A supporting institutional framework, policies, and appetite.
- Processes, staff, and technology that support the program.
- The implementation of the system itself.
The foundation of any successful program is first the institutional framework to support the activity. In the context of AI applications, this will require developing the necessary organizational structure and functions for TSMO. After these enabling actions, the business processes for using AI technologies for TSMO practices will follow more readily and be more effective due to a strong foundation in business processes. These processes will, in turn, ensure that the AI program functions at a high level initially, and continues to adapt and improve as AI technology advances and new applications and functions are identified.
Developing these foundational elements is important and answering the questions in the chapter will help to identify where the strengths and weaknesses lie. It is important to keep in mind in any technology deployment that some of the dimensions are inherently more difficult to deal with than others, yet they all must be addressed to move forward. Failing to consider issues related to staffing and organization, for example, may result in your AI project being a pilot that is never integrated into the main TSMO operation.
A Holistic and Programmatic Approach
The application of AI technologies could be a next step in the provision of mobility and safety services that improve congestion and safety. However, not unlike TSMO strategies, AI applications will likely require new planning and programming approaches, potentially with the need for collaboration with local agencies and other departments of the DOT or agencies outside of the DOT. Agencies might consider coordinating AI application program planning with TSMO program planning. As detailed in previous chapters, the development of AI technologies continues to evolve, and the current state of the art is not yet "plug and play."
Specific AI applications such as fuzzy logic ramp metering and incident detection through closed-circuit television (CCTV) analysis may possibly be considered independently of other programs or agencies. Technologies like automated UASs and digital assistant natural language processing services may benefit from coordination among other programs or agencies. In many long-range planning processes, an "unconstrained" future is envisioned for the planning horizon. That is, if funding was not an issue, what would be built and developed to meet our regional mobility, safety, and environmental goals? How are AI technologies a fundamental element of this future?
After considering an unconstrained future, realistic plans can be considered in the context of funds available for software and hardware procurement, equipment deployment, and operations practices. A similar approach might be applied to AI program planning. A holistic program plan may be developed considering many potential applications and pared back to consider what might be accomplished with more realistic budgets and resources.
Suggestions for How to Approach the Development of Artificial Intelligence Applications
Where to start? Agencies in the early planning stages might take the following steps:
- Convene an interdepartmental workshop to educate stakeholders, partners, and potential partners on AI and brainstorm potential applications and synergies.
- Discuss priorities, opportunities, and barriers to AI applications in each of the TSMO areas.
- Determine a short-list of high-priority applications and a longer list of secondary-priority functions that address regional issues, challenges, and goals. While many goals are generic, tailoring the AI strategy to regional hot-button issues is typically helpful in gaining broader buy-in from decisionmakers and associated agency departments.
- Review the list of general and detailed questions in this chapter and consider the responses of your organization to each.
- Develop a project plan to implement the actions.
The results of the self-assessment process will likely identify a significant list of actions. The next step will be to prioritize the completion of those actions to achieve the desired outcomes within a reasonable schedule. In many program planning activities, it may be helpful to link the completion of key milestones with other related activities. Could the AI technologies be linked to the opening of a new stadium? Reconstruction of a bridge or major interchange? Roll-out of other programs such as managed lanes, Vehicle to Infrastructure (V2I), or Infrastructure to Vehicle (I2V)? Working backwards from a common deadline for other facilities or programs may motivate actions and reveal the schedule for activities that have dependencies on the development of AI software for TSMO.
Relationships of Artificial Intelligence Program Planning with Connected and Automated Vehicles
In 2019, agency planning for connected and automated vehicles is another popular topic for IOOs. The topic of connected and automated vehicles is quite likely to be a point of discussion in AI technology workshops. While most connected and automated vehicles in development by private companies today have some elements of AI, synergies of V2I and I2V services with AI at the TMC may become important in the future. For example, the sheer volume of data that is returned to the TMC by connected vehicles will likely require AI technologies to be effectively processed. Similarly, AI technologies may be helpful in personalizing traveler information back to connected vehicles by fusing agency data (work zones, crashes, traffic congestion, etc.) with vehicle data. Many other synergies will likely be identified in the future as TSMO agencies gain more experience with V2I, I2V, and AI. While there are still many uncertainties regarding timing and availability of both connected and automated vehicles, both technologies and AI are likely to be a significant component of the transportation landscape over the next decade. IOOs considering connected and automated vehicle program plans may find value in considering implications for AI at the TMC and in TMS at the same time.
You may need the AdobeĀ® ReaderĀ® to view the PDFs on this page.
98 "Artificial Intelligence," Wikipedia. Accessed December 10, 2019. [Return to footnote 98]
99 "Capability Maturity Model." Wikipedia. Accessed December 10, 2019. [Return to footnote 99]
100 "Institutional Architectures to Improve Systems Operations and Management." The National Academies of Sciences Engineering Medicine. Accessed January 24, 2020. [Return to footnote 100]