Analysis, Modeling, and Simulation for Traffic Incident Management Applications
Synthesis of Incident Analysis, Modeling, and Simulation Methods
This section describes TIM AMS methods and related applications. In each of the following subsections the current state of the practice is documented. The state of the practice (what is in current use) is discussed separately from the state of the art (that what has been researched).
Survey of Practitioners on TIM AMS Methods
A TIM AMS survey was sent to state departments of transportation (DOT) and metropolitan planning organizations (MPO) in August 2011. The purpose of this survey was to determine current practices in TIM AMS, and to identify areas where practitioners felt that additional guidance would be valuable. The survey questionnaire included eight questions related to TIM:
- Have you ever conducted a study of the incident impacts on congestion (e.g., delay due to incidents)? If so, please attach the relevant study in an e-mail.
- Have you ever conducted a study of secondary crashes due to incidents? If so, please attach the relevant study in an e-mail.
- Do you routinely measure and report secondary crashes?
- What software tools have you either developed or used to estimate incident congestion impacts or secondary crashes?
- In what applications that use incident data does your agency currently engage?
- For which applications would technical guidance be most helpful to you?
- What kind of information/data would be helpful to you in “making the case” for incident management programs internally with your agency?
- In terms of your needs for incident information, what types of technical guidance would help you the most?
Eleven agencies responded by September 2011; they are:
- Delaware Valley Regional Planning Commission (DVRPC);
- Florida DOT, District 6;
- Indiana DOT;
- Kansas City Scout (Kansas DOT and Missouri DOT);
- Maryland State Highway Administration;
- Missouri DOT;
- New Hampshire DOT;
- Regional Transportation Commission (RTC) of Southern Nevada;
- Rhode Island DOT;
- Southeast Michigan Council of Governments; and
- Washington State DOT.
Study of Incident Impacts on Congestion
Out of the 11 agencies who responded the survey, 5 (or 45 percent) indicated that they have conducted studies of the incident impacts on congestion (e.g., delay due to incidents).
Study of Secondary Crashes
Out of the 11 response, 4 (or 36 percent) indicated that they have conducted studies of secondary crashes due to incidents.
Measuring and Reporting Secondary Crashes
Out of the 11 response, 5 (or 45 percent) indicated that they routinely measure and report secondary crashes.
Software Tools for Estimating Incident Congestion Impacts/Secondary Crashes
Out of the 11 response, 8 (or 73 percent) indicated that they have either developed or used software tools to estimate incident congestion impacts or secondary crashes.
Use of Incident Data
Figure 2 shows the applications of incident data by the surveyed agencies. The top five applications are:
- Analysis and evaluation of TIM strategies such as use of service patrols;
- Real-time traveler information dissemination;
- Development and evaluation of TIM plans;
- Agency performance reports; and
- Incident prediction and detection.
Figure 2. Use of Incident Data by Agencies
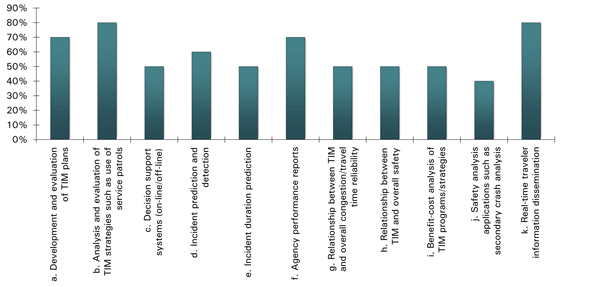
(Source: Cambridge Systematics, Inc.)
Useful Applications of Technical Guidance
Figure 3 shows the useful applications of a TIM technical guidance (with 1 being the most important for TIM AMS application). The top five areas include:
- Relationship between TIM and overall congestion/travel time reliability;
- Development and evaluation of TIM plans;
- Relationship between TIM and overall safety;
- Safety analysis applications such as secondary crash analysis; and
- Analysis and evaluation of TIM strategies such as use of service patrols.
Figure 3. Useful Applications of Technical Guidance, With “1” Being the Most Important
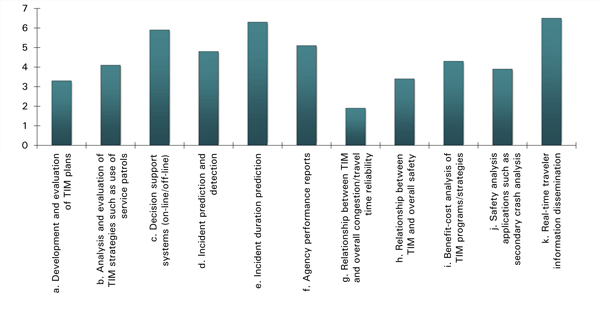
(Source: Cambridge Systematics, Inc.)
Information/Data Helpful for TIM Programs
When being asked what kind of information/data would be helpful for them in “making the case” for TIM programs internally with their agency, the respondents provided the following answers:
- Reliable systemwide speed data.
- Better information related to the benefits of delay/congestion management through transportation systems management and operations.
- Data for reducing congestion and improving safety and linking it with departments such as Operations, Planning, and Safety and Security.
- Injury information is collected for events in which the Service Patrol responds to within the District. But to quantify how many incidents have been averted because of Service Patrol or notification of an incident due to posting of a Dynamic Message Sign (DMS) or through the state 511 system would be helpful information.
- Benefit/cost data, and how incident management is directly tied to safety performance measures.
- Benefit/cost data; find a way to get public officials to understand benefits so they can communicate to media and traveling public; work with upper management so they can present benefit to their board of elected officials.
- Anything would be helpful in justifying operating and maintaining a TMC.
- What can we use to show the cost of secondary crashes and convince our planners and designers to take into consideration traffic safety/congestion/secondary crashes when they design TIM plans for projects.
- Programs to track incident clearance and closure time that can be integrated with control systems.
- Documented safety and congestion improvement results of having a program in place.
Desired Technical Guidance
Figure 4 shows the types of technical guidance that would be helpful. The top two types are technical methods for predicting incident congestion extent (e.g., delay, reliability) and technical methods for predicting incident duration.
Figure 4. Type of Technical Guidance Desired for Improving TIM AMS
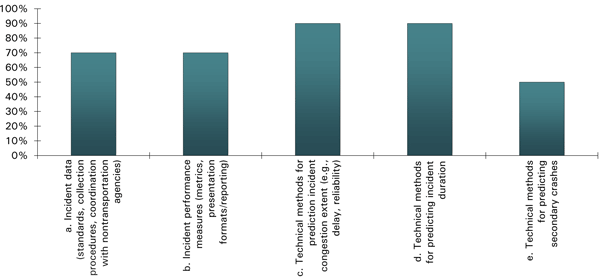
(Source: Cambridge Systematics, Inc.)
TIM AMS Methods
This subsection documents the TIM AMS methods and their applications as revealed through a review of the recent literature as well as through agency contacts. It includes what currently is being used by practitioners and what is available from the research. Five types of approaches to evaluating TIM are described:
- Methods for measuring incident impacts from field data;
- Methods for predicting impacts of a single incident;
- Methods for predicting cumulative incident impacts;
- Methods for predicting incident duration; and
- Methods for predicting secondary incidents.
Methods for Measuring Incident Impacts from Field Data
Methods for measuring in the field the impacts of incidents on delay must address three challenges:
- The definition of what constitutes delay;
- Collection of delay data over extended periods of time; and
- The parsing of the observed delay among incidents and various other possible causes of delay.
Delay is often defined as the difference between the actual travel time and the free flow travel time. Field measurement of travel time over extended periods has historically been difficult, so agencies and researchers have resorted to spot speed measurements over extended lengths of the facility to compute approximate delays.
State of the Practice
Congestion Monitoring Systems
Congestion monitoring systems and programs are either in place or being developed in the major urban areas of the U.S. Monitoring is done using permanent spot speed measurement stations on freeways, targeted field measurements using floating cars of specific facilities, and/or the use of GPS/cell phone tracking devices by commercial vendors of real-time congestion data. However, the assignment of causality to the measured congestion, and the attribution of delay to incidents are extremely rare in current practice. Assignment of causality is more often done as part of specific research efforts.
One example of how an agency defines delay over extended periods is Caltrans. (Caltrans, Mobility Performance Report 2009, February 2011.) Caltrans defines two delay values: the difference between the observed spot speed and 60 mph (free-flow delay), and the difference between the observed spot speed and 35 mph (breakdown delay). The two definitions of delay are used because the agency’s goal is to minimize breakdown delay.
Automated permanent vehicle detector stations approximately a half-mile apart on urban freeways are used by Caltrans to measure five-minute average spot speeds (24 hours per day, 7 days per week). The vehicle-hours of delay measured at each station is the actual volume measured at the station multiplied by the difference in travel times between detector stations at the actual speed and the delay threshold speed (either 35 mph or 60 mph):
Delay = Volume * [(Length/actual speed) - (Length/threshold speed)]
Caltrans currently does not parse the observed delay into various causes, but has plans to do so in future editions of its statewide Mobility Performance Reports.
The TTI 2011 Urban Mobility Report is an example of a national congestion monitoring report which tallies total delay associated with all causes of delay, but does not assign responsibility to any specific causes, such as incidents. Delay is measured by tracking vehicle GPS devices and comparing off-peak to peak travel times.
The Urban Mobility Report: Improved Data Leads to Better Understanding of Congestion
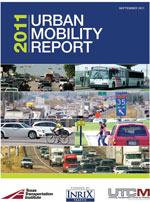
The Texas Transportation Institute has been publishing the Urban Mobility Report (UMR) annually since 1987. Up until 2010, congestion was estimated – rather than measured – using traffic count and roadway characteristics data with analytic methods. Starting with 2010, travel time data collected by a private vendor from GPS-equipped vehicles has been used. As noted in the 2011 UMR:
The new data and analysis changes the way the mobility information can be presented and how the problems are evaluated:
- Hour-by-hour speeds collected from a variety of sources on every day of the year on most major roads are used in the 101 detailed study areas and the 338 other urban areas.
- The data for all 24 hours makes it possible to track congestion problems for the midday, overnight, and weekend time periods.
- A new wasted fuel estimation process was developed to use the more detailed speed data.
- The effect of TIM strategies and other operational treatments on congestion are now considered.
(Source: Texas Transportation Institute.)
State of the Art
Kwon et al. used quantile regression to apportion the causes of measured congestion between incidents and other causes. (J. Kwon, et al., “Decomposition of Travel Time Reliability into Various Sources: Incidents, Weather, Work Zones, Special Events, and Base Capacity,” 2011 Transportation Research Board Annual Conference, Conference CD-ROM, 2011.) In essence, the maximum likelihood contribution of incidents to measured delay is estimated through least squares regression. No underlying traffic behavior model is required.
Skabardonis et al. used a more legalistic approach to separating out incident-related congestion from other congestion. (A. Skabardonis, K. Petty, and P. Varaiya, “Measuring recurrent and nonrecurrent traffic congestion.” In Proceedings of 82nd Transportation Research Board Annual Meeting, Washington, D.C., January 2003.) First the incident logs were consulted to identify nonincident days. These became the baseline congestion days. These days were then compared to days with incidents. The difference in delay between incident days and incident-free days was considered to be the delay associated with incidents.
The statistical and legalistic approaches are somewhat unusual. More typical are traffic model-based approaches such as List et al. which used the classical queuing model in New York State DOT’s Congestion Needs Assessment Model and updated look-up tables of key parameters to estimate the amount of congestion on arterial streets that might be attributable to incidents. (George List, John Falcocchio, Kaan Ozbay, Kyriakos Mouskos, Quantifying Non-Recurring Delay on New York City’s Arterial Highways, Region 2 University Transportation Research Center, New York, New York 2008.) This approach (like all traffic model-based approaches) requires demand volumes during the incidents and the estimated capacity of the facility before, during and after the incident is present. The estimated delays produced by all of the incidents over the year are summed to obtain incident delays for the year.
Methods for Predicting Impacts of a Single Incident
There are a variety of general purpose and incident-specific analytical tools for predicting the delay impacts of a given incident on a given facility. Since the incident is “given,” these models do not focus on predicting the time, location, and type of incident. They focus on predicting the consequences. Incident-specific analytical tools also may predict secondary incidents and the duration of the incident.
General purpose tools include traffic simulation models, and the recently published 2010 Highway Capacity Manual (HCM) method for freeways. Both facility-specific and systemwide impacts of individual incidents can be estimated using microsimulation models, and mesoscopic simulation models. The 2010 HCM currently is limited to single facility applications.
Microsimulation models require more data and will tend to produce more precise estimates of delay effects than mesoscopic models. Mesoscopic models require more precise facility design (and operations) data than demand models, and tend to produce more precise estimates of delay effects than demand models.
Some of the models specifically tailored to the evaluation of incidents can predict incident duration and secondary incident occurrence as well as delay. General purpose models require this information as input and cannot predict these parameters.
State of the Practice – General Purpose Traffic Operations Analysis Models
General purpose traffic operations analysis models are “state of the practice” for the estimation of the delay effects of specific incidents with a given duration. They do not predict incident duration or the probability of secondary incidents.
There are numerous instances in the literature of general purpose traffic operations analysis models being used in research and in practice to predict the delay effects of incidents and incident management strategies. General purpose traffic operations analysis models of specific incidents come in two basic types: Highway Capacity Manual and Simulation (micro and mesoscopic). (See Volumes 1 and 2 of the FHWA Traffic Analysis Toolbox.)
HCM-Based Deterministic Macroscopic Analysis Tools
Overview of Deterministic Tools
Analytic tools predict road traffic capacity, speed, delay, and queuing at intersections and road segments of a variety of types and configurations. Many, though not all, of these tools are based on methodologies published in the Highway Capacity Manual (HCM).
The incident type, start time, location, and duration must be specified. Chapter 10 of the 2010 Highway Capacity Manual then provides special capacity adjustment factors for incidents on freeways. It covers breakdowns and collisions that occur on the shoulders, as well as incidents that block one, two, or three lanes on freeways with two to eight lanes in one direction. The methodology described in Chapter 10 is then used to predict the mean speed (and therefore travel time and delay) and density of traffic on the freeway before, during, or after an incident.
Similar information on capacity and performance effects is not available in the 2010 HCM for urban streets.
What is this tool used for?
HCM-based tools are used to evaluate the effects of traffic operations on isolated transportation facilities such as signalized and unsignalized intersections, freeway mainline segments, freeway weaving segments, freeway ramp merge/diverge areas, and others.
Why is this tool favored for such uses?
The HCM is widely used and its results are accepted (near universally) throughout the industry as representative of actual conditions. Many jurisdictions employ performance standards and thresholds that have been developed with HCM level of service measures in mind.
The HCM is often viewed as the benchmark for evaluating traffic operations. It has a long and storied history of use and acceptance in the industry. Additionally, analysts and decision-makers appreciate the nature of its analytical procedures in terms of consistency of inputs, algorithmic calculations, and resulting output values.
What are challenges and limitations of this tool?
Many users comment that deterministic tools do not properly evaluate the relationships between adjacent or other interacting facilities/control devices, i.e., network affects are ignored. These tools assume demand is fixed and unaffected by highway improvements. Usually the demand model used to forecast demand takes into account approximate effects of highway improvements. The HCM is then used to determine more precise effects for given demand.
Deterministic methodologies are sensitive to the length of the analysis periods. HCM 2000 methodologies do not account for the variation in traffic states within an analysis time period. HCM 2010 freeway methodologies incorporate time slices in a manner similar to macroscopic simulation which addresses variations in traffic states.
Software implementations of the HCM often incorporate default values which make it easy to produce results without giving proper consideration to detailed characteristics of the conditions being evaluated.
When considering the limitations it is important to distinguish between the limitations of the various software implementations and those of the actual HCM. Additionally, certain limitations can be overcome through iterative applications of deterministic tools.
Some deterministic tools do not employ methods documented in the HCM. The comments pertaining to HCM tools apply to these tools for the most part as well, except that these tools are not as widely used and so the likelihood of inconsistency is increased with analysis tools used ‘upstream’ or ‘downstream’ in the analytic process.
What are some software implementations of this tool?
Software examples of deterministic tools include HCS, Teapac, TRAFFIX, RODEL, and SIDRA.
Example Applications:
Skabardonis, A., and M. Mauch, FSP Beat Evaluation and Predictor Models: Methodology and Documentation, Research Report UCB-ITS-RR-2003-XX, University of California Berkeley, 2003 (Updated 2010).
Hagen, L., H. Zhou, and H. Singh, “Road Ranger Benefit Cost Analysis,” Center for Urban Transportation Research, University of South Florida, November 2005.
The first report describes the development and documentation of deterministic spreadsheet-based tools for estimating the benefit/cost ratio of freeway service patrols on a freeway site (“beat”). FSP is an incident management measure designed to assist disabled vehicles along congested freeway segments and reduce nonrecurring congestion through quick detection and response to accidents and other incidents on freeways. The benefits of FSP depend on the beat’s geometric and traffic characteristics, and the frequency and type of assisted incidents. The models, implemented into spreadsheets, calculate the savings in incident delay, fuel consumption and air pollutant emissions based on data that are commonly available to local agency operations staff. The report includes step-by-step instructions for applying the models and analyzing the results. The models have been independently applied by operating agencies in Virginia, Florida, and Hawaii to evaluate the effectiveness of their FSP programs.
Simulation Tools
Simulation Tools: Microsimulation Tools
Microscopic simulation models simulate speed and traffic density by tracking the instantaneous movement of individual vehicles through the network based on a statistical distribution of arrivals and driver behaviors.
The start time, location, lanes blocked, and duration must be specified for each incident. Merging effects are automatically accounted for in microsimulation models; however, distraction effects on the capacity of remaining lanes (rubbernecking) must be specified by the analyst.
What is this tool used for?
As with macroscopic tools, microsimulation tools are used to evaluate changes in operation on a facility that result from changes in demand, capacity, or traffic control. Microscopic tools simulate traffic on a quantum time scale (less than one second) based on the movement and spacing of individual vehicles. Therefore microscopic tools can be used to evaluate the interaction between different vehicles and between vehicles and individual controls and capacity constraints. Furthermore detailed microsimulation models can evaluate the instantaneous and cumulative effects of small changes to facility geometry and timing.
Why is this tool favored for such uses?
Well-calibrated microsimulation tools are superior to other tools for evaluating the sensitivity of operations to small changes. Microsimulation tools allow analysts to identify capacity constraints and opportunities for improvements more precisely. Microscopic tools permit the evaluation of assumptions about driver behavior in addition to management and operational strategies.
Microscopic tools can be incorporated in planning, design, and systems management and provide robust feedback reflecting the cumulative systemwide effects of local modifications and improvements.
Many of the microsimulation tools are packaged with state-of-the-art animation and graphics capabilities. Given the focus of microsimulation tools on individual vehicles, this provides analysts with a convenient and persuasive means of communicating the local and systemwide implications of analysis results.
What are challenges and limitations of this tool?
Microsimulation tools can be prohibitively expensive to implement. The level of detail of a microsimulation model is the direct cause of this. The input data requirements of complex microsimulation models can easily exceed data availability, resulting in the widespread use of defaults. Furthermore, microsimulation models are acutely sensitive to proper calibration and the results generated by the inappropriate use of default inputs can vary considerably from results generated using properly calibrated microsimulation models.
Effective use of microsimulation tools requires a considerable amount of training and quality control. The generation of poor quality analysis is often facilitated by the proliferation of defaults, leading to some confusion over what constitutes calibration.
Microsimulation tools treat origin-destination patterns as fixed inputs. Induced demand is not evaluated though traffic diversion can be evaluated using some microsimulation tools when complete alternate routes are represented within the geographic scope being modeled. Considerable time and training are required for the development of complex models using microscopic tools.
Microsimulation tools require multiple analyses and the results should be averaged. This requirement is due to the variations in results caused by use of random number generators for the starting point of the analysis. Even if the same starting point is used for the analysis, different results may be obtained from the use of different simulation platforms.
What are some software implementations of this tool?
Software examples of Microsimulation tools include CORSIM, VISSIM, SimTraffic, AIMSUN, Paramics, Dynasim, and Transmodeler.
Example Applications:
Chou, C-S, and E. Miller-Hooks, “Exploiting the Capacity of Managed Lanes in Diverting Traffic Around an Incident,” Transportation Research Record: Journal of the Transportation Research Board, #2229, 2011.
Evaluation of the potential benefits and detriments of diverting general traffic into a managed lane when an incident arises along the general purpose lanes using the VISSIM microscopic simulation tool. Continuous and access point diversion strategies were evaluated regarding their impacts on the mobility of general traffic and managed lane users along a concurrent flow lane system on I-270 in Maryland.
Simulation Tools: Mesoscopic Simulation Tools
Mesoscopic simulation models combine properties of both macro- and micro-scopic models.
Many of these models employ multiresolution demand and network modeling with dynamic traffic assignment (DTA) and selected subarea simulation to predict how system performance and traffic demand will vary in response to an incident. The analyst inputs the incident location, capacity reduction, and duration.
What is this tool used for?
Mesoscopic tools are a relatively new addition to the traffic analysis toolbox. Mesoscopic tools combine a focus on individual vehicles and drivers (as with microscopic models) with average measures of speed and density (as with macroscopic models). They are used to evaluate the systemwide effects of changes to driver behavior and performance on individual approaches and segments. Incidents can be directly coded into the network and their effect determined. As a result mesoscopic tools have been recommended for use in planning the operations over citywide or regional networks.
Why is this tool favored for such uses?
They can dynamically assign traffic based on the performance of specific facilities in a network, unlike macroscopic models, but they cannot compare to the precision of microsimulation models, which focus on the behaviors of individual drivers/vehicles.
Mesoscopic models can address a variety of traffic adaptations to network changes, including route shifts and changes in departure times. In the latter sense, mesoscopic tools come closer to addressing induced demand.
Mesoscopic models are easier to use than microscopic models in developing models of large geographic scales. Mesoscopic models are more flexible than macroscopic tools for evaluating different facility types within the same model.
A number of software applications exist to facilitate integration between mesoscopic tools and travel demand models on the one hand, and microscopic tools on the other.
What are challenges and limitations of this tool?
The implementation of dynamic assignment in mesoscopic tools requires a considerable investment in calibration. Minor changes in origin and destination and departure-time patterns can have profound results on the simulated performance of alternative routes.
Mesoscopic tools can provide a misleading level of detail about individual link performance given that they are typically calibrated to generate analysis of large area networks. This is exacerbated by the simplistic representation of signals and other traffic controls.
The sensitivity of mesoscopic tools to default assumptions about driver behavior can be obscured somewhat by the use of average values for link-level vehicle speeds and densities.
Mesoscopic simulation models are more susceptible to failure in reaching convergence or equilibrium due to the additional interaction of dynamic route assignment with the random properties common to many simulation tools.
Considerable time and training are required for the development of complex models using mesoscopic tools.
What are some software implementations of this tool?
Software examples of mesoscopic tools include DYNASMART-P, DYNAST, CUBE Avenue, Dynameq, and TRANSIMS.
Example Applications:
Fei, X., S. Eisenman, H.S. Mahmassani, and X. Zhou, “Application of DYNASMART-X to the Maryland CHART Network for Real-Time Traffic Management Center Decision Support,” Proceedings of the 12th World Congress on Intelligent Transport Systems, San Francisco, California 2005.
Application of DYNASMART-X, a simulation-based real-time network traffic estimation and prediction system based on dynamic traffic assignment (DTA) methodology, to the CHART network in Maryland. The application considers the I-95 corridor network between Washington, D.C. and Baltimore. The CHART network application allows use of the prediction and estimation procedures in conjunction with real-time information to consider multiple traffic management strategies and scenarios in real time. This can improve the ability of the traffic management center to respond to unfolding situations, including incidents, congestion and other unexpected events, through provision of traffic information to travelers and deployment of various control measures. The capabilities and benefits of the system are illustrated through scenario analysis and evaluation that considers real-time information in the context of multiple alternative management strategies in response to the occurrence of an incident on the main traffic facility.
Lili Lou, Examination of Traffic Incident Management Strategies via Multi-Resolution Modeling with Dynamic Traffic Assignment, 2012 Transportation Research Board Annual Conference, Conference CD-ROM, 2011.
Lou demonstrated the use of Dynus-T to model various traffic management strategies during a major freeway crash in the Phoenix region. In their analysis they imported their inputs form the regions travel demand model, conducted analysis in Dynus-T and then exported the output to VISSIM.
State of the Art – Incident-Specific Traffic Operations Analysis Models
Incident-specific traffic operations analysis models are “state of the art,” seeing application primarily in research settings.
The iMiT model is an example of a traffic incident-specific traffic operations analysis model (Khattak). (A. Khattak et al., iMiT: A Tool for Dynamically Predicting Incident Durations, Secondary Incident Occurrence, and Incident Delays, 2012 Transportation Research Board Annual Conference, Conference CD-ROM, 2011.) This tool uses statistical models for incident duration and secondary incident occurrence, and uses a theoretically based deterministic queuing model to estimate associated delays. It has been tested in Hampton Roads, Virginia.
AIMSUN ONLINE is an example of a simulation model designed to support real-time incident management decision-making. (A. Torday, et al., Use of Simulation-Based Forecast for Real Time Traffic Management Decision Support: The Case of the Madrid Traffic Centre, European Transport Conference, 2008.) AIMSUN ONLINE deduces the current traffic status on the streets and the actual demand based on data from permanent detectors. With control plans changing dynamically during the day, AIMSUN ONLINE also reads the current control plan operated at each network intersection. Parallel simulation runs are conducted to assess a variety of possible actions that might be applied in order to improve the network situation compared to the “do nothing” case.
Methods for Predicting Cumulative Incident Impacts
In addition to modeling the effect of a single incident, it also is desirable to know what the cumulative effect of incidents is: this accounts for the variability in incident occurrence and severity that occurs over the course of a year.
The tools for predicting cumulative benefits of incident management fall into three categories:
- Tools that predict the effects of incident management for large systems with minimal details or specifics on incident management methods. These tools are typically sketch planning models.
- Tools that predict the effects of incident management for single facilities with a great deal of detail on the specifics of the incident management methods. These tools are typically microsimulation models but with ongoing advances in Highway Capacity Manual methods, may soon include HCM analysis tools.
- Tools that predict the effects of incident management for multiple facility systems with moderate information on the specifics of the incident management methods. These tools are typically mesoscopic simulators employing dynamic traffic assignment.
Sketch planning models are designed to work at very large geographic scales and forecast the system effects of a variety of traveler information, demand management, capacity, and operational improvements, including incident management.
Overview of Sketch Planning Tools
Sketch-planning tools are typically simple, low-cost analysis techniques, employing highly aggregated and readily available data.
What is this tool used for?
Sketch planning tools are used to provide a quick analytic response to questions about planning concepts and alternatives. Sketch planning tools provide an introduction into the analytic process and can be used to communicate planning relationships and the effects of background trends. Sketch planning tools can be used to rule out scenarios.
Sketch planning tools support experimentation with alternatives and allow for comparisons between large geographic contexts with a minimum investment in set up and analysis.
Sketch planning tools are useful for screening planning alternatives. By incorporating knowledge about cause and effect, and costs-benefits into an automated framework, sketch planning tools offer analytic support for the initial stages of project development with clarity and robustness that surpasses the use of traditional ‘rules of thumb.’
Why is this tool favored for such uses?
Sketch planning tools are inexpensive to develop or acquire. Knowledge of basic policy evaluation concepts and off-the-shelf software makes learning sketch planning tools and applying them easier and less expensive than most other tool types. Sketch tools provide an important benchmark for comparison with subsequent analysis results. It is not the case that sketch planning tools are always wrong and travel demand model analysis is always right when there is a disagreement in their results. Disagreement between sketch planning tools and other tools can be used to prompt a check of the assumptions used with more detailed tools.
What are challenges and limitations of this tool?
Sketch planning tools would benefit from improvements in presentation capabilities. Sketch planning tools often do not generate publishable reports and rarely generate graphical information.
Sketch planning results generally lack precision. Their simplicity is directly related to reliance on a limited number of inputs. The validity of results depends on a constrained range of variation among these inputs, which typically do not extend far beyond the central tendencies established by past experience. Alternatives and scenarios that reflect conditions not measured by the inputs can generate indefensible results. Sketch planning tools are not sensitive to operational features of the project (e.g., signal timing) because they do not represent facilities with resolution.
It should be recognized that the low cost of sketch planning tools might be lost in any tradeoff to enhance their capabilities.
What are some software implementations of this tool?
Software examples of sketch planning tools include HERS, IDAS, SMITE, SPASM, STEAM, and TELUS.
Example Applications:
The Hampton Roads Planning District Commission (HRPDC) MPO used IDAS to quantify the emissions reductions due to reduced incidents as a result of ITS technologies deployed in the Hampton Road region. The IDAS tool was used in combination with the regional travel demand model to estimate the daily incremental emission impacts. The analysis results “showed a substantial decrease in the daily emissions for hydrocarbons (HC) and NOx in the region due to the ITS deployment.
(Source: FHWA Guide on the Consistent Application of Traffic Analysis Tools and Methods.)
Mesoscopic models, HCM methods, and microsimulation models are generally used to predict the impacts of specific incidents, but when combined with scenario generators and applied systematically to a variety of possible incident scenarios, these more computationally intensive tools can produce predictions of the cumulative benefits of incident management.
More information on these types of tools can be found in Volumes 1 and 2 of the FHWA Traffic Analysis Toolbox.
Sketch Planning Tools
Sketch planning models such as HERS IDAS, and TOPS-BC are designed to work at very large geographic scales and forecast the system effects of a variety of traveler information, demand management, capacity, and operational improvements, including incident management. They apply average incident frequencies, average incident durations, and relatively simple speed-flow relationships to estimate systemwide, long-term effects of incidents on system demand and system delay.
Title: Incident Response Evaluation: Phase 3
Objective: This study was intended to improve the understanding of the benefits from Incident Response (IR) actions by Washington State Department of Transportation (WSDOT). The key objectives of this study were to analyze the impacts of incident response service measures on traffic conditions and to develop a methodology to help WSDOT more effectively deploy the Incident Response resources.
Type of Tool/Analysis Used: A variety of statistical analyses of incident data in the Puget Sound region in Washington State were performed to investigate how incidents and incident characteristics affect roadway performance.
Results: For the 2006 study year, a conservative estimate was that crashes and other traffic incidents cost travelers 5,300,000 vehicle-hours of delay, in addition to typical congestion delay, on the Puget Sound region’s freeway system. That was roughly 30 percent of the total delay from all causes that occurred on these roadways. It was recommended that roadway segments (5- to 7-mile stretch) that produce roughly 45 crashes per year in one direction of travel would exhibit enough savings in travel time from incident response to warrant the deployment of incident response on the basis of travel time savings alone. The incident response activities were only financially warranted during times when volumes exceeded a V/C ratio of 0.6 on two-lane (in one direction) roadways or 0.7 on three-lane or larger roads.
HERS
The Highway Economic Requirements System (HERS) is a model for determining optimal highway investment programs and policies. Two versions of HERS exist, one for national-level analyses, the other, HERS-ST is targeted to state DOT-level analyses. HERS does not have a built in network traffic operations analysis module. This information must be provided to HERS from a separate model, such as a travel demand model network or a mesoscopic model network. The traffic operations effects of different investments in incident management programs are modeled off-line and the results input into HERS.
While numerous operations strategies are available to highway agencies, a limited number are now considered in HERS (based on the availability of suitable data and empirical impact relationships). The types of strategies analyzed can be grouped into four categories: arterial management, freeway management, incident management, and travel information. (Highway Investment Analysis Methodology.) For incident management, HERS can evaluate the following strategies for freeways only:
- Incident detection (free cell phone call number and detection algorithms);
- Incident verification (surveillance cameras); and
- Incident response (on-call service patrols).
HERS was used to model incident management effects for FHWA’s 2008 Status of the Nation’s Highways, Bridges, and Transit: Conditions and Performance Report.
More information on HERS.
HERS: The Oregon Experience (U.S. DOT-FHWA Transportation Asset Management Case Studies: Highway Economic Requirements System: The Oregon Experience.)
In 1999, the State of Oregon developed a customized version of the FHWA Highway Economic Requirements System (HERS) tool for use in conducting investment analysis in the State. The State developed the tool so it could develop more credible estimates of user costs and benefits from transportation improvements. When controversy arose in quantifying the costs of delay in a high-profile incident on one of the state highways, the State developed additional postprocessors to produce estimates of “Unexpected Delay” and “Cost of Unexpected Delay” from the HERS-OR tool.
In a major incident that closed a portion of I-5 for 13 hours, a local newspaper cited estimates of user costs that did not match official ODOT estimates from the HERS-OR model. ODOT took the opportunity to develop additional postprocessors to HERS-OR that produced an Unexpected Delay Map and the Cost of Expected Delay that were acceptable and consistent. This information was shared with all departments and the public and now provides a single consistent source for quantifying delay within ODOT.
IDAS
The ITS Deployment Analysis System (IDAS) is software that can be used in planning for Intelligent Transportation System (ITS) deployments. State, regional, and local planners can use IDAS to estimate the benefits and costs of ITS investments – which are either alternatives to or enhancements of traditional highway and transit infrastructure. IDAS can predict relative costs and benefits for more than 60 types of ITS investments. The IDAS ITS components deployed include:
- Incident detection only; and
- Both incident detection and incident response.
IDAS was utilized to model incident management system in Hampton Roads, Virginia. (2008 Conditions and Performance Report.) The results showed a substantial decrease (9 to 14 percent reductions) in the daily emissions for hydrocarbons (HC) and NOx in the region for the two options containing incident management improvements when compared with the control alternative without any improvements.
More information on IDAS.
TOPS-BC
FHWA’s Office of Operations sponsored this project to provide guidance on conducting benefit/cost analysis for operations projects, including incident management. The project developed an Operations Benefit/Cost Analysis Desk Reference as well as software to implement it (TOPS-BC). The benefits of operations projects include those related to travel time reliability. For the impacts of incident management strategies, TOPS-BC uses the IDAS procedures.
SSP-BC
Objective:
The SSP-BC was developed for the I-95 Corridor Coalition and FHWA to fill the need for a comprehensive, cost-effective, and standardized Benefit/Cost (B/C) ratio estimation methodology to facilitate evaluation of existing Service Safety Patrol (SSP) programs throughout the country. The tool is based on commonly accepted assumptions and uses an updateable monetary conversion process. A major strength of the tool is not only its utility for evaluating existing programs, but also its applicability in testing numerous what-if scenarios, including the introduction of a new program or the impact of improvements in service response times.
Methodology:
Data in tables used in the tool were derived directly from simulation run results (travel delays, fuel consumption), regression-based estimates (fuel consumption), a novel hybrid statistical-simulation data methodology with improved model fitness (travel delay), computations (emissions, secondary incidents), and from publically available sources (wages, fuel costs, traffic composition, and monetary conversion rates). Power-based equations that incorporate vehicle characteristics and modal parameters (vehicle mass, velocity, and acceleration) in computing instantaneous power demand for each vehicle type category are used in the estimation of fuel consumption and emissions produced (carbon dioxide (CO2), carbon monoxide (CO), methane (CH4), Nitrogen Oxides (NOx), and Sulfur Oxides (SOx)). The equations are responsive to roadway geometry, traffic volume, grade, and other characteristics of the traffic environment. The output of the tool includes the B/C ratio incorporating user-specified benefit measures, savings in travel delay in vehicle-hours, fuel consumed by passenger cars and light-duty vehicles in gallons, number of prevented secondary incidents, and emission pollutants in metric tons. (E. Miller-Hooks, M. TariVerdi, and X. Zhang, Standardizing and Simplifying Safety Service Patrol Benefit-Cost Ratio Estimation: SSP-BC Tool Development Methodology, Technical Report, I-95 Corridor Coalition, 2012.)
Example Applications
Evaluation of Emissions Impacts of an Incident Management System in Hampton Roads, Virginia
The Hampton Roads Planning District Commission (HRPDC) MPO had invested in deploying ITS technologies in the Hampton Road region. They believed the reduced incidents due to the incident management put in place during the ITS program should logically lead to reductions in emissions. They wanted a tool that could be used to estimate and quantify the emissions reduction. The IDAS software was selected to conduct analysis to quantify the expected emissions reduction. The HDRPC also was interested in quantifying any emissions reductions to the EPA, FHWA, and Air Quality Bureaus to be used in determining the region’s air quality conformity status.
Output runs for a base case and two 2021 scenarios from the regional travel demand model were fed into the IDAS tool for analysis. The IDAS tool was used to estimate the daily incremental emission impacts.
The analysis results “showed a substantial decrease in the daily emissions for hydrocarbons (HC) and NOx in the region for the two options containing incident management improvement when compared with the control alternative.” Run 1 was the current and near-term ITS deployments, while Run 2 represented greater regional incident management, planned for the future. Though EPA did not ultimately use the results it was a first step for HRPDC to begin quantifying the benefits of their incident management program.
CHART
The Coordinated Highways Action Response Team (CHART) is a joint effort of the Maryland Department of Transportation, Maryland Transportation Authority, and the Maryland State Police. Its mission is to improve real-time operations of Maryland’s highway system through teamwork and technology. From February 2001, all incident requests for emergency assistance have been recorded in the CHART information system and this has significantly enriched the available incident data.
The University of Maryland, as part of the ongoing CHART evaluations, developed a predictive equation model based on running experiments with microscopic simulation:
Excess Delay Due to Incidents = e(-10.19 * (V)2.8 * (NLB/TNL)1.4 * (ID)1.78)
Where: TNL = Total number of lanes;
NLB = Number of lanes blocked;
V = Traffic volume; and
ID = Incident duration.
Using this model, it was determined in 2009 that the CHART program reduced delays by 32.43 million vehicle-hours.
More information on CHART.
Title: Benefit-Cost Analysis of Freeway Service Patrol Programs: Methodology and Case Study
Objective: The objective of this study was to estimate the benefits of a Freeway Service Patrol (FSP), the Highway Emergency Local Patrol (H.E.L.P.) program operating in New York State.
Type of Tool/Analysis Used: A CORSIM-based simulation methodology was applied for estimating the benefits of the H.E.L.P. program. The benefits assessed include savings in travel delay, fuel consumption, emissions, and secondary incidents. Using this methodology, the monetary equivalent of these savings was computed to obtain an estimate of the benefit-to-cost (B/C) ratio.
Results: This study showed that the H.E.L.P. program operated with better than two-to-one B/C ratio. If vehicle occupancy, traffic composition with commercial vehicles, and the benefit of avoiding secondary incidents were considered, the B/C ratio could be in a range of 3.4 to 4.2. If considering fatal incidents that were avoided, this ratio would increase to between 13.2 and 16.5.
Scenario-Based Modeling Approaches
Scenario-based modeling approaches apply mesoscopic models, HCM methods, and microsimulation models repeatedly to a variety of possible incident conditions to arrive at an assessment of the cumulative effects of incident management. There are three primary examples of this approach: The Integrated Corridor Management (ICM) analysis, the FHWA ATDM Evaluation Guide, and the SHRP 2-L08 Reliability in HCM project.
Integrated Corridor Management Analyses
The evaluations of Integrated Corridor Management strategies for Minneapolis, Dallas, and San Diego used travel demand models, mesoscopic simulation models and microsimulation analysis in combination with selected incident scenarios (Table 1). The analysis of multiple incident and weather scenarios (where a variety of incident types can occur at multiple times and locations) were strictly limited to manage analysis costs.
The analysis found significantly positive benefit/cost ratios for integrated corridor management strategies, which include incident management.
This analysis approach can evaluate a wide variety of incident management strategies but it requires a significant investment in analysis effort for the various models that must be employed.
More information on the ICM.
Table 1. ICM TIM Modeling Tools
Model Type |
Minneapolis |
Dallas |
San Diego |
Regional Travel Demand Model |
Metro model in TP+ |
NTCOG model, TransCAD |
TransCAD |
Mesoscopic Simulation Model |
Dynus-T – supported by University of Arizona |
DIRECT – supported by Southern Methodist University |
None |
Microscopic Simulation Model |
None |
None |
Transmodeler/Micro |
Source: Adapted from: ITS Research Success Stories.
FHWA ATDM Evaluation Guide
The FHWA Active Transportation and Demand Management Evaluation Guide (ATDM Guide) and future replacement for Chapter 35 of the 2010 Highway Capacity Manual currently is under preparation. The ATDM Guide recommends the creation of three different prototypical incident scenarios (no incident, one lane blocked, two lanes blocked) for each of good weather and bad weather days for a total of 6 capacity scenarios. The 6 capacity scenarios are each matched with 5 different levels of demand. The result is 30 scenarios for evaluating incident management and other ATDM strategies.
Special demand, capacity and speed adjustment factors currently are being developed to reflect the effects of incidents and various incident management strategies on these factors. The method will be sensitive to traveler information strategies, speed control strategies (VSL), and lane management strategies (temporary shoulder lane use, etc.).
Once the scenarios have been created and the demand/capacity/speed adjustment factors computed, conventional HCM methods are then used to evaluate facility performance.
The HCM predicted performance for each scenario is weighted by the probability of the scenario occurring over the course of a year to obtain average, median, and any desired percentile (e.g., 95th percentile) result.
Software to implement aspects of the ATDM methodology is being developed. The proposed ATDM evaluation methodology is being tested on the I-15 corridor in San Diego.
SHRP 2 Projects Relevant for Incident AMS
Several completed and ongoing SHRP 2 projects deal specifically with the prediction of travel time reliability, of which incident impacts are a major component. These SHRP 2 projects are discussed below.
More information on this methodology can be obtained from SHRP 2 staff.
SHRP 2-L08 Incorporation of Reliability in the HCM
The SHRP 2-L08 Reliability Analysis Guide for the Highway Capacity Manual (Reliability Guide) currently is under preparation. The Reliability Guide will recommend the creation of several hundred to several thousand demand, weather, and incident scenarios to predict future travel time reliability distribution.
Two methods for generating scenarios are being considered. One enumerates all possible scenarios and selects the ones of most interest for more extensive evaluation. The other method uses a Monte Carlo approach to generate the scenarios.
Special capacity and speed adjustment factors currently are being developed to reflect the effects of incidents (but not incident management strategies) on these factors. The SHRP 2-L08 project is focusing on predicting existing and future reliability under existing control conditions, rather than predicting how changes in operational strategies can affect reliability.
Once the scenarios have been created and the demand/capacity/speed adjustment factors computed, conventional Highway Capacity Manual (HCM) methods are then used to evaluate facility performance.
An improved HCM Urban Streets method is being developed to better support reliability analysis on arterial streets. The improved method will be able to account for the impacts of queues on upstream signal operation.
The HCM predicted performance for each scenario is weighted by the probability of the scenario occurring over the course of a year to obtain average, median, and any desired percentile (e.g., 95th percentile) result.
Software to implement the methodology is being developed. The proposed methodology will be tested on a half dozen freeway and urban street data sets.
SHRP 2 L03 Analytical Procedures for Determining the Impacts of Reliability Mitigation Strategies
SHRP 2 Project L03 produced two types of statistical equations based on empirical data for predicting reliability measures. The first set relates the mean congestion condition, as measured by the travel time index (TTI) to a variety of reliability metrics. A strong correlation was found between the mean and the rest of the reliability metrics used, including standard deviation, upper percentiles of the travel time distribution, and on-time measures. The second set relates reliability metrics to demand, capacity, incident blockage, and weather. Publication of the report is expected in late 2012.
Methods for Predicting Incident Duration
When an incident occurs, the timely estimate of its duration plays a key role in the overall incident management process. Reliable incident duration predictions can help traffic managers in providing correct and essential information to road users, applying appropriate traffic control measures at or near the incident location and evaluating the effectiveness of the incident management strategies implemented. The duration of an incident can have several definitions, depending on what is chosen for the start and end times of the incident. Generally the start time is when the incident is first detected by incident management personnel. Ideally the start time would be the time when the incident actually occurred, but this cannot be known with certainty. However, in urban areas, the time between actual start and detection is very small because most incidents are reported by travelers via cell phones within a very short time of the actual occurrence. The end time is usually selected as the time when all lanes are open to traffic or when the last responder has left the scene.
State of the Practice
In Maryland, a Rule-Based Tree Model (RBTM) was applied to develop the prediction model for freeway incident duration by Kim et al. (W. Kim, S. Natarajan, and G. Chang, Analysis of Freeway Incident Duration for ATIS Applications, 15th World Congress on Intelligent Transport Systems and ITS America’s Annual Meeting, 2008.) The model was developed based on the Maryland State Highway (MDSHA) incident database. The overall confidence for the estimated model was over 80 percent. In cases where RBTM did not provide incident duration within a desirable range, a discrete choice model was developed as a supplemental model.
State of the Art
There are several methods that have been developed in predicting incident duration, as listed below:
- Regression model;
- Hazard-based duration regression model;
- Log-logistic model;
- Prediction/decision tree model;
- Support/relevance vector machine model; and
- Bayesian network model.
Methods for Predicting Secondary Crashes
Secondary crashes are associated with vehicles in close proximity due to a queue formed from a primary incident, the abrupt “end-of-queue” condition caused by a primary incident, collisions with emergency vehicles and personnel, and rubbernecking in both the current and opposite directions of travel. Secondary crashes can be severe, especially at night when visibility is reduced and traffic queues are unexpected. Modeling methods to predict secondary crashes would be greatly enhanced if traffic management center personnel could flag crashes that occur in the queue caused by the primary incident or from opposite direction rubbernecking. Currently, researchers must derive these items analytically.
State of the Practice
Researchers at the Virginia Center for Transportation Innovation and Research also developed a dynamic queue-based tool to identify primary incidents (Secondary Incident Identification tool – SiT). They have used SiT and iMiT together to begin to improve the state of the art in modeling secondary incidents. (A. J. Khattak, X. Wang, H. Zhang, and M. Cetin. Primary and Secondary Incident Management: Predicting Durations in Real Time. Final Report VCTIR 11-R11, Virginal Center for Transportation Innovation and Research, April 2011.)
State of the Art
The following is a list of methods that have been developed and used for quantifying the occurrence and characteristics of secondary crashes.
- Regression model;
- Ordered logit model;
- Probit model;
- Logistic regression model;
- Bayesian network model; and
- Simulation-based secondary incident filtering method.
For instance, a study conducted by Zhan et al. used a comprehensive incident database on I-95 from District 4 of the Florida DOT to identify freeway secondary crashes and their contributing factors. (C. Zhan, A. Gan, and M. A. Hadi. Identifying Secondary Crashes and Their Contributing Factors, In Transportation Research Record: Journal of the Transportation Research Board 2102, 2009.) A method based on a cumulative arrival and departure traffic delay model was developed to estimate the maximum queue length and the associated queue recovery time for incidents with lane blockages.
Vlahogianni et al. also recently utilized neural networks and statistical approaches to study the impact of weather on secondary crashes. (E. I. Vlahogianni, Matthew Karlafits and Foteini Orfanuo., Modeling the Effects of Weather on the Risk of Secondary Incidents, 2012 Transportation Research Board Annual Conference, Conference CD-ROM, 2012.) Their findings were that speed, volume, number of blocked lanes and vehicles involved in crash significantly influence the probability of a having a secondary incident.
A compendium of how transportation agencies are dealing with secondary incidents can be found in the document: Traffic Incident Management Performance Metric Adoption Campaign.
Title: Primary and Secondary Incident Management: Predicting Durations in Real Time
Objective: The main objectives of this study were to analyze the occurrence and nature of secondary incidents in the Hampton Roads (HR) area in Virginia, and develop tools that can analyze primary and secondary incidents at the planning and operational levels.
Type of Tool/Analysis Used: A dynamic queue-based tool, Secondary Incident Identification Tool (SiT), was developed to identify primary and secondary incidents from historical incident data. An on-line tool, iMiT, was developed to predict the remaining duration of an existing incident, the chances of a secondary incident based on the characteristics of the primary incident, and the associated delays.
Results: This study found that secondary incidents account for nearly 2.0 percent of Transportation Operations Center (TOC)-recorded incidents, using the 2006 data. Of all accidents, 7.5 percent had associated secondary incidents, 1.5 percent of disabled vehicles had secondary incidents, and 0.9 percent of abandoned vehicles had secondary incidents. The average duration of secondary incidents in Hampton Roads was 18 minutes, which was 4 minutes longer than the mean duration of other incidents, indicating that secondary incidents were not necessarily minor “fender benders.” The study also found that a 10-minute increase in primary incident duration was associated with 15 percent higher odds of secondary incidents.
Predicting Incident Characteristics (Independent Variables)
Incident models are built using indicators of incident performance as the predictor variables (e.g., incident duration, lane-hours lost due to incidents). Knowing how TIM strategies affect these independent variables is therefore of utmost importance. A number of studies have been done over the past two decades that can be used for this purpose. SHRP 2 L03 assembled the most recent studies in this area.
You may need the Adobe® Reader® to view the PDFs on this page.