Analyzing Travel Time Reliability: Data and Analysis Methods
Printable version [PDF 1.3 MB]
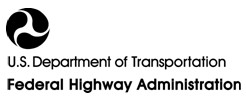
U.S. Department of Transportation
Federal Highway Administration
Office of Operations
1200 New Jersey Avenue, SE
Washington, DC 20590
FHWA-HOP-22-086
July 2023
Travel time reliability is important to travelers for several reasons:
- Travelers have less tolerance for unexpected delays. To compensate for unexpected delays, travelers will plan for unreliable travel by “padding” their schedule to avoid being late.
- Reliability has costs for users:
- Economic competitiveness for freight movements is linked to uncertain travel times.
- Studies place variability costs at about the same level as those for average travel time.
- Reliability can be treated by addressing roadway “events,” thus highlighting the benefits of Transportation System Management and Operation (TSMO).
Travel Time Reliability Key Points
Over the past decade, travel time reliability has emerged as a key concept for assessing and managing the performance of the transportation system. Simply put, travel time reliability describes how personal mobility changes from day-to-day for trips made at the same time. Savvy commuters understand that travel times for their work trips, which tend to occur at roughly the same time every weekday, can vary based on a variety of conditions they encounter. Figure 1 shows that the interaction of disruptive events—traffic incidents, inclement weather, special events, and work zones—with daily demand, physical capacity, and traffic controls lead to different travel time experiences on different days. Travel time reliability describes the nature of this variability in travel times in terms of the quality, consistency, predictability, timeliness, and dependability of traveler experiences on the transportation system.
Figure 1. Travel time reliability is the result of seven interacting factors related to congestion.
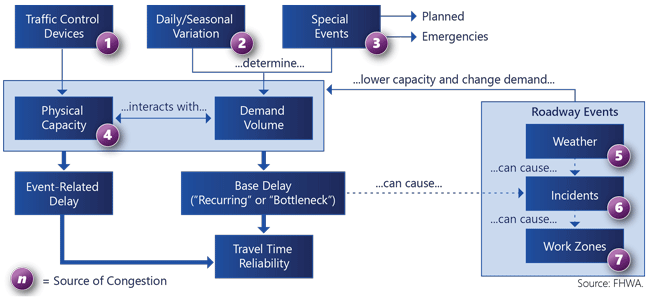
(Source: FHWA.)
Travel Time Reliability Performance Measures
A suite of travel time-based measures has been established over the years. These measures all describe the variability or uncertainty of travel times in some way. Table 1 shows common reliability measures in use by transportation agencies.
Table 1. Typical travel time reliability performance measures definitions.
Measures |
Descriptions |
Core Measures |
Planning time index (PTI) |
95th percentile travel time index (TTI)—95th percentile travel time divided by the free flow travel time. |
80th percentile TTI |
80th percentile TTI—80th percentile travel time divided by the free flow travel time. |
Semi-standard deviation |
The standard deviation of travel time pegged to free flow travel time rather than the mean travel time—variation is measured relative to free flow travel time. |
Failure/on-time measures |
Percentage of trips with average speed less than 50 mph, 45 mph, and 30 mph.
Reliability rating: Percentage of trips serviced at or below a threshold TTI (1.33 for freeways, 2.50 for urban streets). |
Supplemental Measures |
Standard deviation |
Usual statistical definition. |
Misery Index (modified) |
The average of the highest 5 percent of travel times divided by the free flow travel time. |
Measuring Travel Time Reliability: Measurements Versus Processed Information
Numerous data sources have been used to develop travel time reliability performance measures. These data all represent the travel time of individuals or vehicles to different degrees. The gold standard of data sources for reliability is currently vehicle trajectory data available from private vendors who process Global Positioning System (GPS)-derived times and locations from personal devices. These data represent the passage of individual travelers in space and time as they use the transportation system. As such, the data provided to analysts are actual measurements and offer the greatest resolution for potential applications. Other data sources for reliability either represent aggregated statistics, are limited in their geographic scope, or both, and include the following:
- Vehicle trajectory data are often aggregated to average travel times for 1-minute or longer time intervals over discrete roadways links.
- Roadway-based sensing of toll tags, Bluetooth® devices, and license plates provides actual travel times, but is limited to the locations of the sensors.
- Freeway detectors measure speeds at a point, not travel times over a distance, and are aggregated before being communicated from the field and archived.
Measuring Reliability With Different Data Sources
This project undertook a series of tests where reliability measures were computed for the same roadway and time period using different data sources. The project specified the processing steps, assumptions, and definitions necessary to transform high-resolution data into reliability measures. Among these measures were the following: free flow speed calculation, spatial and temporal aggregation, and outlier identification. The key findings of the data source comparisons are as follows:
- Because trajectory data measures the paths of individual vehicles, the travel time distributions display high variability in travel times compared to data from vehicle probes and detectors (figure 2). Since these data are the basis for developing travel time reliability measures, it is clear that the type of data used will influence the measures. Both vehicle probe and detector data are temporarily pre-aggregated (5-minute intervals for this study). Vehicle-to-vehicle variation from trajectory data is useful in the context of operations strategies, many of which are aimed at “smoothing” traffic flow.
- As a result, reliability measures developed from trajectory data are generally higher in value than those developed from pre-aggregated probe data. This result is probably due to the inclusion of vehicle-to-vehicle variations described above. However, the result indicates that the two sources should not be mixed for congestion monitoring purposes as it could result in misleading results.
- Reliability measures developed from pre-aggregated probe data via different processing methods are reasonably close in values. Two processing methods were tested: the snapshot method and the virtual probe method. For relatively short urban facilities and trips, either method may be used; however, no strong reason exists to go through the extra calculation complexity of the virtual probe method. Within the broader context of congestion monitoring and performance reporting, pre-aggregated travel time data (at least at 5-minute intervals) is adequate to capture macroscopic congestion conditions.
- Reliability measures developed from roadway detector data are almost always lower in value than those developed from pre-aggregated probe data based on data from Los Angeles, CA, and San Francisco, CA, detectors.
- All the reliability measures tested in the study were correlated with each other. The mean TTI, 80th percentile TTI, and the PTI were strongly correlated with each other and loosely correlated with the semi-standard deviation and the level of travel time reliability metric as defined by Performance Measure Rule 3 (System Performance, Freight, and Congestion Mitigation and Air Quality). The former relationships could be useful in planning applications requiring that reliability measures be developed from model-developed average conditions.
- Reliability measures for long distance trips are more sensitive to the pre-aggregated vehicle probe data-processing method than for urban facilities. When trips are considered that are made continuously throughout the year, the difference in processing method is generally around 5 percent, although trips exposed to a high number of urban conditions show more deviation. When discrete time periods are considered, the deviation is greater. This result leads to the use of the virtual probe method to develop reliability measures for long-distance trips rather than the snapshot method.
- The third performance measure rule (PM3) system reliability measure generally decreases as PTI increases for freeways, which in theory should be the case, although there is much scatter in the data. The difference most likely lies in the nature of the measurements. System reliability relies on a threshold to determine if a facility is unreliable (binary), whereas PTI is a continuous variable. With binary variables, one facility may be only slightly over the threshold while another might be way over the threshold. In both cases, the facility is deemed to be unreliable, but the latter case is clearly more severe, a condition captured by PTI but ignored by the system reliability measure.
- On signalized arterials, the correlation between the PM3 system reliability measure and PTI is extremely low. In general, the measures indicate that travel is more unreliable on signalized arterials (higher PTI values), but this result may be a scaling issue. PTI is determined by assuming a free flow or ideal travel time. On signalized highways, free flow speed is often set based on the midblock speed (for example, in the Highway Capacity Manual (Highway Capacity Manual 6th Edition: A Guide for Multimodal Mobility Analysis. Washington, DC: The National Academies Press). This definition was used initially, but accounting for this adjustment, signalized arterial reliability is still routinely higher than for freeways.
Figure 2. Speed distribution comparison for different data sources, I–695 in Maryland (5-mile section).
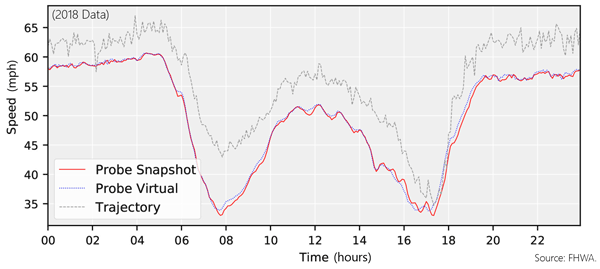
(Source: FHWA.)
Future Efforts for Travel Time Reliability
Moving forward, the following issues bear further investigation:
- Data processing procedures—including establishing consistent benchmarks for reliability measures; quality control, especially for emerging data sources; and simplified procedures for the initial processing of vehicle trajectory data.
- Trip-based reliability—because of the nature of the data that have been available, nearly all reliability reporting is based on the facility perspective. However, vehicle trajectory data from vendors are now becoming available that allow trip-based measures to be developed. A comprehensive mobility measurement program will involve using both trip- and facility-based measures because they both inform analysts about the nature of mobility in a region. Trip measurement includes factors in addition to congestion exposure—how users interact with entire landscapes which are driven by land use patterns, demographics, and economic forces. Finally, trip measurement is the starting point for measuring accessibility, which assesses the degree to which residents have access to different types of opportunities. To promote trip-based reliability performance, additional work is needed to define fixed or multiple paths for a trip, the most useful measures for trip reliability, and how to integrate trip reliability into general planning (e.g., congestion management process) and evaluations of completed projects.