Behavioral/Agent-Based Supply Chain Modeling Research Synthesis: Data Challenges
Printable Version [PDF 1.1 MB]
You may need the Adobe® Reader® to view the PDFs on this page.
Contact Information: Operations Feedback at OperationsFeedback@dot.gov
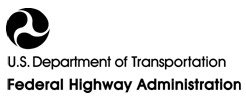
U.S. Department of Transportation
Federal Highway Administration
Office of Operations
1200 New Jersey Avenue, SE
Washington, DC 20590
FHWA-HOP-18-006
March 2018
BACKGROUND AND CHALLENGE
Acquiring the data and finding the resources required to support a behavioral supply chain model is often challenging, given the privacy and confidentiality issues surrounding supply chain data. Many data sources exist for estimating, calibrating, validating, and forecasting a freight modeling system. Advanced freight travel demand modeling in the United States use publicly available data sources to model freight movements such as the Freight Analysis Framework (FAF)1. Publicly available data sources are often sufficient for applying the behavioral supply chain models, but not for development, calibration or validation, where additional data sources are needed.
DATA AVAILABLE FOR MODEL INPUTS AND ESTIMATION
Behavioral supply chain freight models typically require the following eight types of model input and estimation data:
-
Zone systems for behavioral supply chain models are tiered Transportation Analysis Zone (TAZ) systems so that the model can operate at a national (with limited international zones), regional or statewide scale.
-
Network systems represent multimodal networks supporting the movement of goods. Typically, modal networks include highway and rail; more advanced models also include water and air.
-
Employment data are developed at the TAZ level using locally sourced employment datasets, often derived from the quarterly census of employment and wages. In addition, the County Business Pattern (CBP) offers marginal distributions of employment by size and industry at the county level.
-
Transfer facilities include intermodal terminals, warehouses, and distribution and consolidation centers. Data for transfer facilities locations can be found in the National Transportation Atlas Database (NTAD) merged with data on employment from the TAZ-level employment dataset.
-
Economic data represent the value of commodities exchanged between industries, also called Input/ Output (IO) Make and Use Tables. Economic growth rates are also required for forecast models.
-
Freight flows are developed from the Commodity Flow Survey (CFS)2 and products like the FAF provide a useful processed and cleaned dataset of freight flows sorted by mode at the national scale. These data must first be disaggregated to the local level. Including a procurement market model that produces freight flow forecasts based on economic and infrastructure forecasts in the freight model introduces more transparency and sensitivity into the process.
-
Freight Surveys can include commodity flow surveys, establishment surveys, truck diary surveys, vehicle use surveys to estimate parameters for mode, shipment sizes, distribution channels and buyer-supplier matching.
-
Truck Global Positioning System (GPS) Data is used to estimate parameters for truck time of day models and vehicle origin-destination patterns.
Data for estimating behavioral supply chain freight model parameters requires disaggregate data, which is difficult to obtain. Data from national, state, or regional commercial vehicle surveys is difficult and costly to collect. Thus, these data are collected infrequently or with small sample sizes. National surveys commonly used for model estimation of behavioral supply chain models include the following:
- CFS is collected every five years (1997, 2002, 2007, 2012) and includes a large sample size. Unfortunately, these data are not available in disaggregate form [the 2012 CFS Public Use Microdata (PUM)3 can been explored as an option for model estimation, but there are issues with data suppression].
- The Freight Activity Microsimulation Estimator (FAME) survey4 (2009–11, in three waves) is an establishment survey with a small sample, but it contains information on transfer facilities, mode, and commodity that have supported estimation of distribution channel models.
- The Vehicle Inventory and Use Survey (VIUS)5 was last collected in 2002, but is still being used as the best source for truck payload factors.
Several states and regional transportation agencies have conducted establishment surveys, but only the establishment surveys that are combined with commercial vehicle diary surveys can be used effectively to estimate model parameters for behavioral supply chain models. Passively collected GPS data offer a partial solution to the challenge of collecting data on commercial vehicles. GPS data typically includes data on travel time, origin-destination, and time of travel. Private vendors (e.g., American Transportation Research Institute, Streetlight) offer large samples of GPS data with these data and some vendors (i.e., EROADS, INRIX) provide additional attributes on commercial vehicle travel, such as truck type, commodity or industry group, and weight.
DATA FOR MODEL CALIBRATION AND VALIDATION
Travel demand modeling best practice includes selecting different data sources for model calibration and validation than those used in model estimation. This practice has not always been possible given limited data availability for the development of behavioral supply chain freight models. Available data sources identified for model calibration and validation typically fall into five categories:
-
Freight Surveys are used for shipment sizes, distribution channels and freight flows. These are typically collected locally by the agency developing the behavioral supply chain model, but could also be conducted as national surveys.
-
Freight Flow Data is a publicly available national source from the 2012 CFS PUM file, which is used to compare the distribution of shipment sizes by commodity and modal freight flows.
-
Truck GPS Data are used to compare truck trip distributions, time of day, and volumes and is available through private vendors for a specific State or region.
-
Weight Data ais a publicly available national source from Weight In Motion (WIM) stations or VIUS surveys, which are used to adjust vehicle loading factors used to convert shipment tonnages to truck trips.
-
Modal Volumes includes truck counts, Public Use Waybill Sample for rail, and T-100 data for air, which are used to compare observed and modeled volumes, Waterborne Commerce statistics or Port Import/Export Reporting Service (PIERS) data for water. These are used to develop calibration weights for the mode and transfers model and the estimation of import and export volumes for each port.
If multiple datasets are available, then the best practice is to select one dataset of a single type for model estimation and a second dataset for model calibration and validation.
Table 1. Primary Data Sources by Modeling Needs and Availability.
Data Source
|
Availability (latest available)
|
Spatial
|
Temporal
|
Modes
|
Industry Detail
|
Commodity Code
|
Model Inputs
|
Use
|
County Business Pattern
|
Public, 2014
|
County
|
Annual
|
N/A
|
Two to Six-digit NAICS6 codes
|
N/A
|
✓
|
Model Estimation, Calibration and Validation
|
Bureau of Economic Analysis Input/Output Accounts
|
Public, 20157
|
National
|
Annual
|
N/A
|
Two to six-digit NAICS6 codes
|
N/A
|
✓
|
Model Estimation
|
FAF
|
Public, 2015
|
FAF Zone
|
Annual8
|
All modes
|
N/A
|
Two-digit SCTG9 Commodities
|
✓
|
Model Estimation, Calibration and Validation
|
CFS
|
Public, 2012
|
BEA11 Zone
|
Every five years
|
All modes
|
N/A
|
Two-digit SCTG9 Commodities
|
N/A
|
Model Estimation, Calibration and Validation
|
Vehicle Inventory and Use Survey (VIUS)
|
Public, 2002
|
State
|
Every five years
|
Truck
|
N/A
|
Two-digit VIUS Commodities
|
N/A
|
Model Estimation and Calibration
|
TRANSEARCH
|
Private, 2015
|
County
|
Annual
|
All modes
|
N/A
|
Four-digit STCC10 Commodities
|
N/A
|
Model Calibration and Validation
|
Surface Transportation Board Waybill
|
Public, 2014
|
BEA Zones
|
Annual
|
Rail
|
N/A
|
Four-digit STCC10 Commodities
|
N/A
|
Model Calibration and Validation
|
DATA AND RESOURCE ASSESSMENT
There are multiple potential data sources used by agencies to estimate, calibrate, and validate the forecasting of a freight modeling system. Table 1 summarizes primary data sources used for behavioral supply chain freight models and includes details on each data source. The table does not include observed data (e.g., truck counts, WIM data) or local survey data available from local agencies.
DATA PRIVACY AND SHARING ISSUES
The internal and external sharing of data is crucial to most business operations. It forms the basis for most business decision-making processes and models. Conversely, the protection of this data, which is often proprietary in nature, is essential to reducing both personal and professional risk and liability. The data management landscape has changed greatly over the last decade. Technological advances associated with collecting business information have been exponential, leading to a massive increase in the amount of data that is generated, stored and distributed. Maintaining business confidentiality and data privacy is a well understood necessity for firms competing in a free market.
Data privacy and sharing issues include:
-
Vehicle-Level Data Issues. Data privacy concerns at the individual vehicle level derived from vehicle- or component-generated data, such as GPS devices, recorders, and embedded communications systems.
-
Macro Supply Chain Data Issues. Supply Chain data typically includes inventory level, sales data, order status for tracking and tracing, sales forecasts (and/or other forecasts) and production/delivery schedules.
-
Government Data Sharing: Friend or Foe? Datasharing with government is both an opportunity and concern. The public sector’s objectives to improve freight mobility and efficiency require access to real-world, near-real-time freight data, but the public sector has few tools, nor compelling reasons to protect that same critical data from “inappropriate uses or distribution”.
-
New Data Partnerships: The Clear Solution. New arrangements and data-sharing tools are needed to create and foster the data-sharing partnerships that could lead to improved freight systems.
FOR MORE INFORMATION
Learn more about the SHRP2 program, its Capacity focus area, and Freight Demand Modeling and Data Improvement (C20) products at www.fhwa.dot.gov/GoSHRP2/
The second Strategic Highway Research Program is a national partnership of key transportation organizations: the Federal Highway Administration, the American Association of State Highway and Transportation Officials, and the Transportation Research Board. Together, these partners conduct research and deploy products that help the transportation community enhance the productivity, boost the efficiency, increase the safety, and improve the reliability of the Nation’s highway system.