Roadway Network Productivity Assessment: System-Wide Analysis Under Variant Travel Demand
Final Report
The analysis documented in this report examines the hypothesis that the system-wide productivity of a metropolitan freeway system in peak periods is higher in moderate travel demand conditions than in excessive travel demand conditions. The approach in this effort characterizes system-wide productivity by aggregating link-level speed and traffic volume (count) data. The study utilized 2007 Los Angeles and Chicago traffic data (both flow and speed) archived as part of the Urban Congestion Report (UCR) databases. In addition, an extensive archive of incident, work zone and weather data were available to identify the underlying conditions related to congestion patterns and bottleneck locations in the network. System-wide travel demand, delay and productivity were estimated for two Mondays in September-October 2007. One day was the worst congested Monday (September 10 2007) during the two-month period and the other Columbus Day (October 8 2007). Our a priori expectation was that travel demand on Columbus Day would be lower than on September 10 2007 since government and other workers receive a paid holiday on Columbus Day. Examining differences in uncongested link-level flow rates at five-minute periods throughout the day, we estimated that over the entire day travel demand is slightly lower on Columbus Day when compared September 10 2007 (0.5% in Los Angeles, 1.3% in Chicago). A more significant reduction in estimated travel demand was estimated in the AM peak (6-9 AM), however (1.6% in Los Angeles, 9.9% in Chicago). Incidents, weather, and work zone conditions were comparable on both Mondays in both cities. Several measures of productivity based on a combination of flow and speed data were developed to capture system-level efficiencies of the freeway networks. Overall daily system delay, compared to the worst Monday, is reduced by more than 35% in both Los Angeles and Chicago, with larger delay reductions in the AM peak period. Aggregate system-wide uncongested flow rates are higher on Columbus Day (19% in Chicago, 33% in Los Angeles) in the AM peak period. The study suggests that higher system-level productivity and efficiency can be observed when travel demand is observed to closely match overall system capacity, resulting in less frequent onset and reduced duration of freeway breakdown conditions. By reducing demand and preventing congestion from taking hold, demand management through pricing or other mechanisms could recover the daily waste of time and drop in freeway system productivity that occurs on congested highways when traffic flow breaks down.
KEYWORDS: Performance Measurement, Throughput, Reliability, VMT, Los Angeles, Chicago, System Performance, Bottleneck Analysis.
Since 2002, Noblis has processed travel time and traffic volume data from urban freeway systems to calculate three key network performance measures (hours of congestion, travel time index, and planning time index) to provide a national snapshot of urban mobility on a monthly basis. This national snapshot is reported as a part of the Urban Congestion Report (UCR) effort in a series of graphical dashboards illustrating congestion trends and factors that contribute to congestion: weather, work zones, incidents and variations in travel demand.
In collecting, assuring quality control, and analyzing these data, Noblis has developed a large repository of traffic volume and speed data for more than twenty U.S. cities and metropolitan areas. In 2007, this data repository was used to quantitatively analyze the key components of traffic congestion for the Chicago metropolitan area (Hardy et al., 2007). One element of this analysis examined area-wide aggregations of vehicle miles traveled (VMT) at five-minute periods on weekdays. These VMT data were correlated with average network speed over the same five minute intervals. In this congested network, the relationship between system-level aggregated VMT and speed suggested that an overall system maximum productivity could be observed when average network speeds operated in a relatively narrow range. Based on the results of that study, FHWA tasked Noblis to conduct additional research on the characterization of system-level productivity under a range of estimated travel demand and network speed conditions.
The analysis documented in this report examines the hypothesis that total freeway system peak period productivity is higher in moderate travel demand conditions than in excessive travel demand conditions. The Noblis analysis addressed the hypothesis using two approaches:
- A micro-level study examining interactions between individual bottlenecks on the urban roadway network that characterizes travel demand and productivity relationships on the “edges” of congestion evolving throughout the day; and
- A macro-level study using aggregated measures for the entire urban roadway network system to examine changes in system-wide productivity measures for various travel demand conditions.
Noblis conducted these studies using archived UCR speed and traffic volume data for roadway networks in Los Angeles and Chicago metropolitan areas. This report summarizes the macro-level study of aggregate system productivity in UCR networks from Los Angeles and Chicago.
The focus of this study was to characterize efficient operations in a multi-link network utilizing time-variant aggregations of link-level speed and traffic count data. The study examined the capability of these particular data to support a range of quantitative productivity measures. In general, we sought measures that characterize the productive output of the roadway system over time. In addition, we sought productivity measures that would support meaningful comparison between different time periods, both within a day and between days. Given a particular measure or approach, overall network productivity was estimated to test the study hypothesis:
System-level productivity in a roadway network with multiple bottlenecks is highest when travel demand is moderate and close to some optimal threshold, but declines when excessive demand causes disruption to network-wide operations.
The study hypothesis is illustrated graphically for within-day variation in Figure 1 in the lower graph representing an excessive demand condition for the roadway system. The x-axis represents the time of day around a peak period where the network is initially lightly loaded, e.g., 12 AM – 3 PM. System-level travel demand (dashed line) and productivity (solid line) are plotted on the y-axis. In the early, lightly-loaded period, travel demand is lower than some system maximum. No congestion at bottlenecks form and system productivity rises with increased travel demand. When the demand for travel rises above some threshold, congestion begins to form at individual bottlenecks, reducing the volume of vehicles passing through the bottlenecks. These individual bottlenecks experience lower link-level productivity in a congested state. System-level productivity is also reduced since the component link-level bottlenecks serve fewer vehicles in any five minute time period.
At this critical point, desired demand for travel exceeds system capacity and the two plotted lines in the excessive demand graph begin to diverge. In fact, as demand continues to rise in the peak period, additional bottlenecks form and congestion between bottlenecks begin to interact, further reducing the capacity of the system to deliver vehicles along the network efficiently. This causes overall system productivity to decline throughout the peak period. After the peak period has ended, the system must continue to process the remaining inventory of vehicles even as new demand drops off. This may cause congestion at bottlenecks to dissipate and system level productivity to rise. Peak system-level productivity and system-level utilization will be observed close to the breakdown point where travel demand and system-level productivity measures diverge.
In comparison, under more moderate demand conditions (illustrated in the upper graph in Figure 1), desired demand for travel closely approaches but does not greatly exceed the roadway system maximum productivity. The result is little overall system productivity loss throughout the peak period. If one compares the total network productivity within the peak period, the total productivity in the moderate demand case will be higher than in the excessive demand case.
To examine this hypothesis, multiple system-level measures were developed using archived speed and link-level vehicle-miles-traveled (VMT) data from the Los Angeles and Chicago metropolitan areas from late 2007. These system-level measures differ from traditional point measures of capacity or volume because they characterize a comprehensive network-wide capability of a roadway system to serve vehicular demand, rather than capability to deliver higher vehicle counts at a single location on a specific facility.
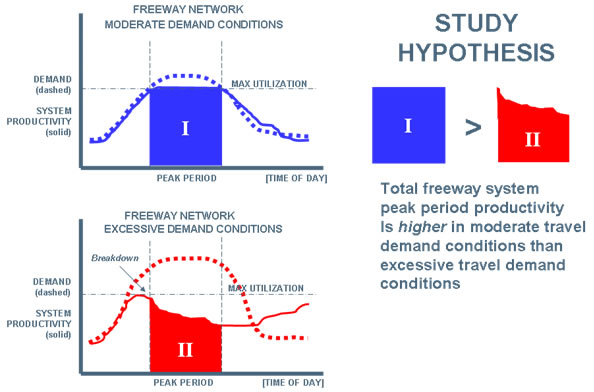
Figure 1. Study Hypothesis, Peak Period Productivity Under Moderate Demand versus Excessive Demand
Given the high levels of recurrent congestion in the Los Angeles and Chicago metropolitan areas, these regions were selected as test-beds to consider the study hypothesis. The studies utilized 2007 traffic data (both flow and speed) archived as part of the Urban Congestion Report (UCR) databases in both metropolitan areas. In addition, an extensive archive of incident, work zone and weather data were available to identify the underlying conditions related to congestion patterns and bottleneck locations in the network.
The Los Angeles and Chicago UCR networks are illustrated in Figures 2 and 3. These are two of the largest roadway networks in the UCR archive, with over 1,200 directional miles of coverage in Los Angeles and over 590 miles in Chicago. The Los Angeles network is divided into 153 links of an average length of 7.9 miles. The Chicago network is similarly subdivided into 75 links with an average length of 7.9 miles. The UCR archive contains a link-level VMT at five minute intervals as well as an average link speed for each five minute time interval. These estimates are generated from combining station-level data from the multiple sensor stations along the links.
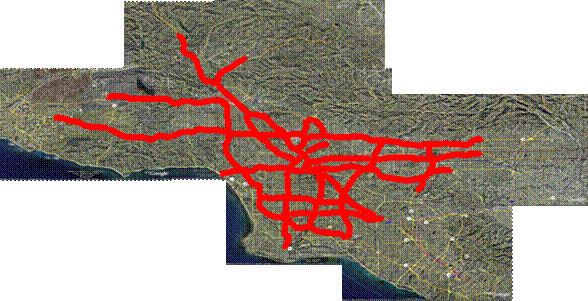
Figure 2. Los Angeles UCR Network
Noblis considered a range of days from the UCR archive to identify low, medium and high travel demand days. To choose the appropriate days to be used for comparisons, Noblis analyzed the daily VMT level (VMT estimates by link every 5 minutes) and speeds for the entire system for September and October 2007. The results showed that Mondays had the most congested conditions; i.e., lowest aggregate daily network speeds. Based on this analysis, the following three days were identified for comparison:
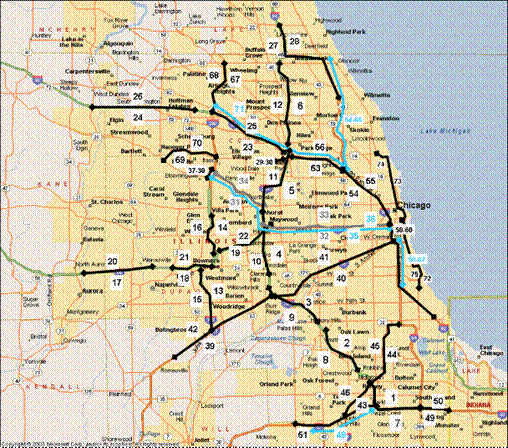
Figure 3. Chicago UCR Network
- Low Demand Day—Labor Day, a national holiday falling on a Monday where most businesses and government offices are closed (September 3, 2007).
- Medium Demand Day— Columbus Day, a federal holiday falling on a Monday where government offices are closed but most businesses remain open (October 8, 2007).
- High Demand Day—A non-holiday Monday from identified in the months of September and October 2007 with the lowest aggregate network speed of all non-holiday Mondays (September 10, 2007).
For all three days in both cities, data relating to work zones, incidents and weather conditions were identified. Weather was similar in all cases. Although work zone and incident records did differ by each of these days, there were no significant differences in the aggregate number of these disruptions. Further, there were no major incidents (freeway closures or crashes blocking lanes of major freeways for more than 30 minutes in the peak period). Work zone activity remained nearly constant throughout the two month period, that is, no new work zones were put in place during peak periods between Labor Day 2007 and Columbus Day 2007.
In this analysis, VMT and speed data are utilized at five minute intervals throughout the entire 24-hour period. In both cities, the link-level VMT and speeds in the UCR archive are calculated using a weighted combination of multiple fixed location sensor stations along the link. These data are used to characterize the aggregate system level demand, total delay in the system, and estimate overall system productivity using a variety of measures. This section describes how these key measures are calculated using the UCR data in both Los Angeles and Chicago.
First, let us examine the fundamental speed and count data obtained for the UCR project to better understand the nature of the underlying data. Figure 4 presents sample link level data from a bottleneck along northbound I-405 in the Los Angeles network. These data are taken from three Mondays in September-October 2007 (Labor Day, Columbus Day, and a typical congested Monday 9/10/2007). Each data point in the figure represents a 5-minute speed and volume link-level estimate combining data from multiple sensor locations along the roughly 10-mile long link. The result is the familiar backward bending speed flow relationship with high-speed travel maintained up to some critical link capacity. For this link, both high-speed and high-volume flow can be observed at around 50 mph. The observed pattern of speed and volume is consistent with expectations from both the HCM and other analyses of freeway traffic flow. When the link becomes congested, both speed and flow decline. Many other bottleneck links with similar properties can be found across both the Chicago and Los Angeles networks, although the critical breakpoints for speed and volume vary. This relationship confirms the fundamental data in the analysis contains the bottleneck effect at the link-level.
When the link carries traffic volume near a critical capacity with high speed, we characterize the productivity of the link as being higher than in the cases where congestion causes a drop to both speed and carrying volume. In addition, one can also argue that in low-volume, high-speed conditions, the link itself could carry additional volume without breakdown and is underutilized compared to the high-volume and high-speed case. Therefore, in any five minute period, one way of characterizing the productivity of the link would be to examine the volume of vehicles traversing the link. One could also characterize productivity by looking at the volume of vehicles traversing the link in a high-speed or uncongested state.
Figure 5 shows the same segment of I-405 plotting VMT (average weighted volume by sensor along the link multiplied by link length) against time of day for a congested (typical) Monday. Note that peak volume in Figure 4 (or VMT in Figure 5) is reached after 6:00 AM and then declines sharply (27%) during the AM peak period. Link-level VMT only recovers this loss in the mid-day period. Note that the VMT data in Figure 5 are also color-coded by speed to indicate congestion levels. The green portions of the figure indicate five-minute intervals where speeds are 50 mph or greater. The yellow (speeds between 25 mph and 50 mph) and red (slower than 25 mph) segments show lower speeds associated with congestion and the low productivity regime associated with breakdown conditions.
Figure 4. Speed/Volume Relationships Observed on Northbound I-405 ( Los Angeles),
Three Mondays in September-October 2007
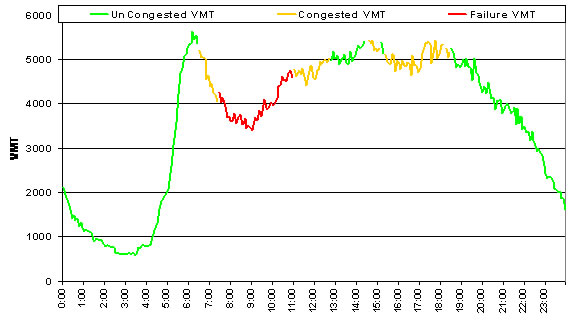
Figure 5. VMT Classification by Time of Day, Northbound I-405 ( Los Angeles), September 10 2007
The aggregation of link-level data into system-wide measures should fairly reflect contributions from all links in the system. To do this, we chose to aggregate link-level VMT data rather than average link volumes to weight longer links higher than shorter links. This helps to reduce the impact of arbitrary network definition on measures. For example, simply breaking a longer link into three pieces would triple the amount of impact from that section of the system if a VMT measure had not been utilized.
As we discussed earlier in this section, one method of characterizing productivity we examined was to aggregate link-level VMT across all links in the network. However, this total VMT aggregation showed little difference between significantly different network conditions. One example appears in Figures 6(a) and 6(b) comparing September 10 (High Demand) and October 8 (Medium Demand) in Los Angeles. Both days have a similar VMT time-of-day pattern, but significantly different AM peak speed patterns. Our observations of the data indicate that there is a self-regulating component to a connected, but open system like a freeway network. In a closed system, a link-level bottleneck may develop and cause a reduction in volume that can be observed downstream. In an open system, with many access points, additional volume can fill downstream of the bottleneck with little apparent loss of productivity. Queued vehicles held upstream of a bottleneck are converted into increased volume (and productivity) downstream from the bottleneck. For this reason, although aggregate VMT is one way of characterizing system productivity, it does not reflect the quality of travel in the system.
One analogy is the consideration of two factories, each of which produces 100 widgets per day. The first factory has a reject rate for its widgets of 50% while the other has a reject rate of 1%. By simply comparing the number of widgets produced (100 vs. 100), we do not fairly represent the inherent higher productivity realized by the second factory (50 vs. 99). To reflect the quality of travel in the system, we may choose to characterize productivity as the total amount of VMT operating above the critical link capacity across the network.
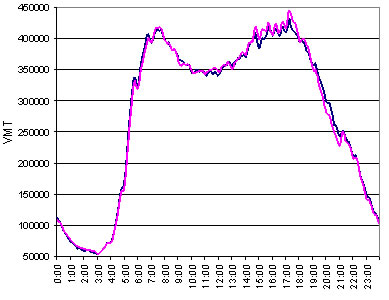
Figure 6. System-Wide VMT and Speed Comparison ( Los Angeles),
High and Medium Demand Days
Figure 6. System-Wide VMT and Speed Comparison (Los Angeles), High and Medium Demand Days Figure 6 consists of two line graphs showing aggregate VMT and speed by time of day. Figure 6.a compares system-wide VMT of September 10 (High Demand) and October 8 (Medium Demand) in Los Angeles and shows that both days have a similar VMT time-of-day. Figure 6.b compares system-wide average speed by time of day with significantly different AM peak speed patterns.
Figure 6. System-Wide VMT and Speed Comparison (Los Angeles), High and Medium Demand Days Figure 6 consists of two line graphs showing aggregate VMT and speed by time of day. Figure 6.a compares system-wide VMT of September 10 (High Demand) and October 8 (Medium Demand) in Los Angeles and shows that both days have a similar VMT time-of-day. Figure 6.b compares system-wide average speed by time of day with significantly different AM peak speed patterns.
Classification of VMT. To bring this notion of travel quality into the analysis, we utilized five-minute link-level speed estimates to differentiate between over utilization and underutilization conditions. Because the identification of breakpoints for each link in both large networks was out of scope for this effort, a set of speed breakpoints were established for three categories of link-level productivity for each city:
- Uncongested VMT: Average speeds > 50 mph (LA), 45 mph ( Chicago)
- Congested VMT: Average speeds 25-50 mph (LA), 22.5-45 mph ( Chicago)
- Failure VMT: Average speeds < 25 mph (LA), 22.5 mph ( Chicago)
Total System-Wide Uncongested VMT was used in both networks as one measure of system-wide productivity. The aggregation is a simple summation of link-level VMT meeting the uncongested criterion established above at five-minute intervals throughout the day. VMT associated with links operating in the congested or failure regimes are not included in the system-level calculation.
Aggregate System-Level Travel Demand Estimation. Our study hypothesis is based on the a priori assumption that aggregate travel demand will be lowest on Labor Day and highest on the High Demand day, and that Columbus Day demand will be somewhat less than on the High Demand day. There is evidence that such an assumption has merit. For example, a Society of Human Resource Management research of 2007 holiday schedules (SHRM, 2007) shows that 94% of organizations close their offices on Labor Day, while only 11% do so on Columbus Day. Further, both Labor Day and Columbus Day are federal holidays, and federal employees have a paid holiday. Most state and local government offices are also closed on Labor Day, while policies with regard to Columbus Day are less clear-cut. Census data from 2000 indicates that federal, state and local government workers represent 14.6% of the total workforce in California; in Illinois the figure is 12.7%. Whether these figures are clearly indicative of reduced total travel demand is a subject for debate. Simply having a day off does not mean that workers do not travel – in fact, one may argue total travel demand could possibly be increased on Labor Day reflecting return traffic from the long holiday weekend during the afternoon period.
In order to quantify the change in travel demand at the system level, another key element in this study is the interpretation of volume and speed data to characterize travel demand. At the link level, the VMT data in the UCR database always reflects traffic counts and link length, but may not always describe the travel demand for the link.
We cannot determine travel demand from VMT data in the case where a link is in a congested condition. Instead, we estimate travel demand by looking at differences between days on links during times of day when we can confirm the link is operating in an uncongested condition for both days. We assess link condition based on the speed criteria used to classify VMT (above). The result is that, for this study, travel demand differences between two days (e.g., Columbus Day and the High Demand Monday) are made as pair-wise comparisons. Such a comparison is shown in Table 1. The results indicate that demand over the entire day is slightly lower (0.5-1.6%) but that demand in the AM peak period is more significantly reduced (1.6-9.9%). This makes some intuitive sense because people are likely to defer travel to later in the day on holidays compared to normal work days.
Table 1. Percentage of Government Workers by State, and Travel Demand
Differences Estimated Between Columbus Day and September 10 2007
Percent of Employees (2000 Census Data)
Government Employees |
California |
Illinois |
Local |
8.3% |
6.8% |
State |
4.0% |
3.8% |
Federal |
2.3% |
2.1% |
Total |
14.6% |
12.7% |
Noblis Demand Estimate from UCR Date |
Los Angeles |
Chicago |
AM |
-1.6% |
-9.9% |
PM |
1.4% |
-0.6% |
Entire Day |
-0.5% |
-1.3% |
System-level Delay Estimation. Another key productivity measure that reflects quality of travel is system delay. In this study, system level delay is defined as travel times on links lower than the posted speed limit. Because we lack speed limit data for each link in the UCR archive, we estimated the posted-speed as the closest 5 mph interval that falls below the observed 85th percentile travel speed in uncongested conditions. This approximation has been demonstrated to be a relatively accurate (plus/minus 5 mph) when compared against posted speed data at selected locations in both the Chicago and the Los Angeles networks. Aggregate system level delay in each five minute period is calculated as the VMT-weighted sum of all link level delay in that time interval.
This section presents results from the study in both Los Angeles and Chicago. First, a pair-wise comparison of system productivity on Columbus Day compared to the High Demand Monday is summarized for each city. Second, a more detailed examination of aggregate VMT classified by speed within a day for the Los Angeles network is presented. Some observations about a critical value for maximum system-level uncongested VMT is explored across the set of three Mondays in Los Angeles. Finally, this observation is converted into a time-variant maximum system capacity figure and a quality-weighted productivity ratio calculated. This methodology is extended to the Chicago network and results presented and compared.
Over the period from 5 AM – 10 PM traffic delay is reduced by almost 40 percent on Columbus Day. In particular, AM peak (6-9 AM) travel delay is reduced by more than 47%. PM peak travel delay (4-7 PM) also declines by over 37%. Figure 7 plots system-level delay by time of day. Over the entire day, uncongested VMT is 14% higher on Columbus Day than on the High Demand Monday, 33% higher in the AM peak, and over 18% higher in the PM peak. Total VMT (regardless of speed) across the entire day differs by less than 0.05%, with similarly small differences in both peak periods as indicated in Table 1.
Figure 7 plots system-level delay by time of day. The X-axis represents time of day and the Y-axis represents the hours of delay for entire network. The red line plots the system-level delay of the High Demand Monday, September 10, 2007 and the blue line plots the system-level delay of Columbus Monday, October 8, 2007. AM peak (6-9 AM) travel delay is reduced by more than 47%. PM peak travel delay (4-7 PM) also declines by over 37%.
Figure 7. System-Level Delay Comparison, Los Angeles, Columbus Day and September 10 2007
Columbus Day vs. High Demand Monday: Chicago Results
Over the period from 5 AM – 10 PM traffic delay is reduced by over 35 percent on Columbus Day. In particular, AM peak (6-9 AM) travel delay is reduced by more than 45%. PM peak travel delay (4-7 PM) also declines by over 51%. Over the entire day, uncongested VMT is 14% higher on Columbus Day than on the High Demand Monday, 19% higher in the AM peak, and over 49% higher in the PM peak. Total VMT (regardless of speed) across the entire day differs by less than 0.03%, with a similarly small difference in the PM peak period, as indicated in Table 1.
The results obtained in Los Angeles and Chicago in the pair-wise examination of system-level productivity generally support the study hypothesis when travel quality is considered. When total system VMT is considered without consideration for travel quality, both days are similarly productive. System delay or speed-classified aggregate VMT show a significant difference between the two days, however. Delay is reduced by 35-40% while uncongested VMT rises roughly 14% in both networks.
System-Wide Uncongested VMT Delivered By Time of Day: Los Angeles
One consideration within the analysis was to seek evidence that some analog of the link-level critical capacity (or productivity) could be found at the system-level. To pursue this idea, we examined system-wide aggregated uncongested VMT patterns by time of day in Los Angeles over the three Mondays.
Results of this analysis are shown in Figures 8-10. The presentation of the data is analogous to that of Figure 5, where link-level VMT is displayed by time-of-day and color-coded by speed level. In Figures 8-10, however, we are reporting VMT from many links in both networks. At any point in time throughout the day, the network may have component links in any one of the three speed-depended classification regimes (uncongested, congested and failure). Total VMT is plotted against time-of-day (blue line), summing over all VMT in the system regardless of classification. In addition, however, the VMT in the system in each of the three speed classifications are also plotted (green, yellow and red lines).
One noteworthy element of Figures 8-10 can be observed by a consideration of uncongested VMT (green line). Figure 8 shows results for Labor Day, our lowest demand Monday. One can see that nearly all of the VMT throughout the day in Los Angeles is in the uncongested regime except for some moderate congestion between 3-10 PM. A system-level limit of 300-350K VMT per five minute period seems to be roughly a point where uncongested VMT begins to be converted to congested VMT on Labor Day.
Similarly, if we consider Figure 9 (High Demand) we see breakpoints for uncongested VMT that are close to the limits on Labor Day. The first breakpoint occurs prior to 6 AM at around 300K VMT per five minute period. The system never fully recovers to a completely uncongested state until the mid-day, but system-wide uncongested VMT reaches 350K per five minute period just prior to the PM peak (2-3 PM). In Figure 10 (Medium Demand – Columbus Day) a similar pattern can be observed, with a slightly higher peak in the afternoon above 350K uncongested VMT per five minute period.
Figure 8. System-Wide VMT Classification by Time of Day, Los Angeles, Low Demand Monday, Labor Day 2007
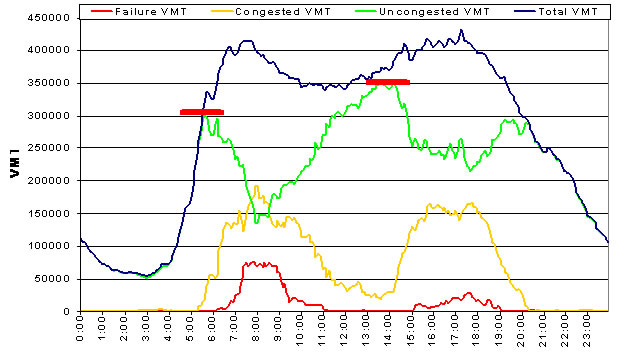
Figure 9. System-Wide VMT Classification by Time of Day, Los Angeles, High Demand Monday, September 10 2007
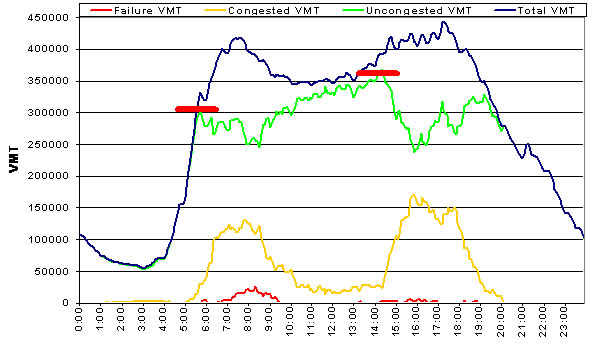
Figure 10. System-Wide VMT Classification by Time of Day, Los Angeles, Medium Demand Monday, Columbus Day 2007
The maximum level of uncongested VMT the system is capable of moving in a five-minute period appears to have analogous properties to the link-level relationship between speed and flow. Up to roughly some level, the system can accept additional travel demand without any components undergoing breakdown and congested (low-flow, low-speed) conditions. Clearly this level depends on many factors: e.g., weather, incidents, and variations in travel demand (both in terms of total demand but also the distribution of demand across the network).
Does higher system-wide uncongested VMT indicate higher system-level productivity? After all, total VMT (regardless of classification) continues to climb in the peak periods even after the observed breakpoints for uncongested VMT are observed. In both Figures 9 and 10, total VMT peaks later in the peak periods at levels roughly 25% higher than at these critical break points. From a vantage point that is indifferent to travel quality, these uncongested VMT breakpoints are less critical. However, one can argue that if the notion of system-wide productivity includes consideration of travel quality, then peak system-wide uncongested VMT does represent a peak in system-wide productivity.
If one accepts that maximum system-level uncongested VMT is one way of quantifying a quality-weighted measure of network-wide productivity, then one may want to look across a large number of days to find conditions under which this measure is maximized. An analysis of the Los Angeles data looking across every day in the two-month period September-October 2007 is presented in Figure 11. Uncongested VMT for our familiar trio of Labor Day, Columbus Day and September 10 2007 are plotted by time of day. In addition, the thick green line represents a composite day built up from finding the highest uncongested VMT found in any five-minute time slice across the 61 days considered from September 1-October 31, 2007. It is likely that incident delays were minimal during these times.
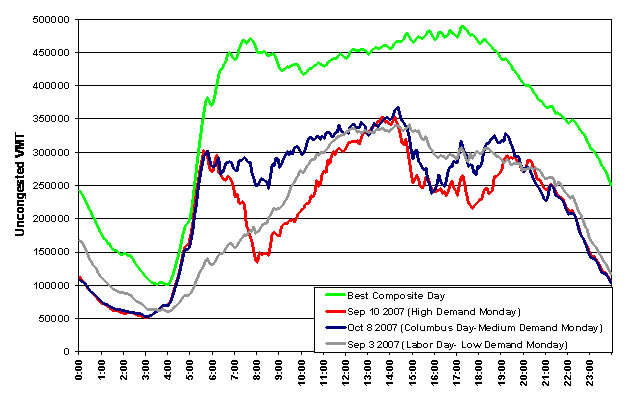
Figure11. Maximum System-Wide Uncongested VMT by Time of Day, Los Angeles High, Medium and Low Demand Days
One observation is that the uncongested VMT observed in any five-minute time slice from the composite day is always higher than any uncongested VMT value obtained in any of the three Mondays we considered in our detailed analysis. This implies that there was no five minute time slice in our three Mondays that represented a maximum level of quality-weighted productivity seen over the two-month period. At some times of day (early AM peak period), particularly for Columbus Day and September 10 2007, system-level uncongested VMT approaches the composite day.
Clearly, the amount of uncongested VMT that can be loaded onto the Los Angeles network is highly dependent on the underlying travel demand pattern. This includes notions of geographic distribution in demand, as well as temporal distribution. In particular, different days of the week are observed to have different times most likely to reach peak uncongested VMT. This peak occurs later in the morning on Fridays, and in the mid-afternoon period on weekends. Because of this, and the possibility that incident delay may be minimal at some times randomly, the composite probably does not represent an achievable goal for any particular day when one manages a freeway system. However, it can be useful as a uniform measuring stick to characterize quality-weighted system productivity and to provide a measure when comparing two different days.
To explore this concept, we define the quality-weighted productivity ratio (QPR) as the level of uncongested VMT carried by the system in any five minute time slice compared against a composite day comprised of the maximum uncongested VMT observed in that same time slice across many days. Figures 12-13 show results using the ratio in Los Angeles and Chicago.
Figure 12. Quality-Weighted Productivity Ratio by Time of Day, Los Angeles, High, Medium and Low Demand Mondays, 2007
Figure 13. Quality-Weighted Productivity Ratio by Time of Day, Chicago, High, Medium and Low Demand Mondays, 2007
In Figure 12, Columbus Day is shown to have the highest QPR in the AM peak (0.62). On Labor Day, the system does not see sufficient demand to fully load the network in the AM peak (QPR 0.40). During the congested High Demand Monday, the system is overloaded during the AM peak and the QPR is lower than on Columbus Day (0.47). In the PM peak, Labor Day posts the highest QPR (0.63), better than on Columbus Day (0.61) and September 10 2007 (0.52). Columbus Day has the highest QPR when the 5 AM – 10 PM (All Day) period is considered. Similar results are obtained when data from Chicago are considered (Figure 13). Columbus Day has the highest All Day QPR and AM peak QPR, while Labor Day has the highest PM peak QPR.
Other observations of note regard the extreme values of QPR in both networks observed over the three days studied in each city. In Chicago , a minimum QPR of 0.2 can be observed at 6 AM on Labor Day, while the highest QPR of 0.93 occurs later that same day between 8-9 PM. In Los Angeles, the lowest QPR of 0.3 is observed at 8 AM on September 10 2007. This low for Los Angeles is only a short time after the peak QPR of 0.87 is reached just before 6 AM.
ne can argue that when the QPR is near 1.00 the system has the most productive balance of travel demand and overall system carrying capacity. Certainly these conditions are the most desirable for travelers and system managers – the highest level of vehicles operating at conditions above link-level breakdown conditions and minimal system-level delay.
Results from the analysis support the study hypothesis, with a single important caveat. When system productivity is defined using measures like delay or quality-weighted VMT, then it is clear that higher productivity is linked with conditions that best match overall system demand with system carrying capacity. The QPR measure appears to be the best single measure in this study to illustrate this concept. The QPR drops as low as 0.2 when travel demand is far lower than system carrying capacity, as in at 6 AM in Chicago on Labor Day. It also drops to as low as 0.3 when excessive demand has caused broad network congestion as at 8 AM in Los Angeles on September 10 2007. High QPR values can be found during the AM peak on Columbus Day or in the latter portions of the Labor Day PM peak period when networks are loaded close to some level where a large volume of vehicles can be accommodated without significant breakdown.
The caveat from the study is that the hypothesis is less well supported when measures of productivity that are indifferent to quality of travel are considered. For example, Total VMT (regardless of speed) does not indicate a significant difference between Columbus Day and September 10, 2007 in any peak period or when the entire day is considered. Our observations of these measures indicate that there is a self-regulating component to a connected, but open system like a freeway network. In a closed system, a link-level bottleneck may develop and cause a reduction in volume that can be observed downstream. In an open system, with many access points, additional volume can fill downstream of the bottleneck with little apparent loss of productivity. Queued vehicles held upstream of a bottleneck are converted into increased volume (and productivity) downstream of the bottleneck.
One analogy that may be helpful when considering productivity measures is the consideration of two factories, each of which produces 100 widgets per day. The first factory has a reject rate for its widgets of 50% while the other has a reject rate of 1%. By simply comparing the number of widgets produced (100 vs. 100), we may not be fairly representing one kind of higher productivity realized by the second factory (50 vs. 99).
The QPR and other measures provide a new and potentially insightful way of considering the productivity of a large complex system like a freeway network. Maximum uncongested VMT appears to be a useful measure in characterizing overall system resilience and carrying capacity. Either measure could be used to systematically identify productivity losses associated with incidents, work zone activity, weather or other impacts if these other data were combined with the concurrent speed and traffic count data.
To view PPT files, you need the Microsoft PowerPoint Viewer.